Thematic Analysis: A Step by Step Guide
Saul McLeod, PhD
Editor-in-Chief for Simply Psychology
BSc (Hons) Psychology, MRes, PhD, University of Manchester
Saul McLeod, PhD., is a qualified psychology teacher with over 18 years of experience in further and higher education. He has been published in peer-reviewed journals, including the Journal of Clinical Psychology.
Learn about our Editorial Process
Olivia Guy-Evans, MSc
Associate Editor for Simply Psychology
BSc (Hons) Psychology, MSc Psychology of Education
Olivia Guy-Evans is a writer and associate editor for Simply Psychology. She has previously worked in healthcare and educational sectors.
On This Page:

What is Thematic Analysis?
Thematic analysis is a qualitative research method used to identify, analyze, and interpret patterns of shared meaning (themes) within a given data set, which can be in the form of interviews , focus group discussions , surveys, or other textual data.
Thematic analysis is a useful method for research seeking to understand people’s views, opinions, knowledge, experiences, or values from qualitative data.
This method is widely used in various fields, including psychology, sociology, and health sciences.
Thematic analysis minimally organizes and describes a data set in rich detail. Often, though, it goes further than this and interprets aspects of the research topic.
Key aspects of thematic analysis include:
- Flexibility : It can be adapted to suit the needs of various studies, providing a rich and detailed account of the data.
- Coding : The process involves assigning labels or codes to specific data segments that capture a single idea or concept relevant to the research question.
- Themes : Representing a broader level of analysis, encompassing multiple codes that share a common underlying meaning or pattern. They provide a more abstract and interpretive understanding of the data.
- Iterative process : Thematic analysis is recursive, not linear. Researchers move back and forth between phases, refining codes and themes as their understanding of the data evolves.
- Interpretation : The researcher interprets the identified themes to tell a compelling and insightful story about the data.
Many researchers mistakenly treat thematic analysis (TA) as a single, homogenous method. However, as Braun and Clarke emphasize, TA is more accurately described as an “umbrella term” encompassing a diverse family of approaches.
These approaches differ significantly in terms of their procedure and underlying philosophies regarding the nature of knowledge and the role of the researcher.
It’s important to note that the types of thematic analysis are not mutually exclusive, and researchers may adopt elements from different approaches depending on their research questions, goals, and epistemological stance.
The choice of approach should be guided by the research aims, the nature of the data, and the philosophical assumptions underpinning the study.
1. Coding Reliability Thematic Analysis
Coding reliability, frequently employed in the US, leans towards a positivist philosophy . It prioritizes objectivity and replicability, often using predetermined themes or codes.
Coding reliability TA emphasizes using coding techniques to achieve reliable and accurate data coding, which reflects (post)positivist research values.
This approach emphasizes the reliability and replicability of the coding process. It involves multiple coders independently coding the data using a predetermined codebook.
The goal is to achieve a high level of agreement among the coders, which is often measured using inter-rater reliability metrics.
This approach often involves a coding frame or codebook determined in advance or generated after familiarization with the data.
In this type of TA, two or more researchers apply a fixed coding frame to the data, ideally working separately.
Some researchers even suggest that some coders should be unaware of the research question or area of study to prevent bias in the coding process.
Statistical tests are used to assess the level of agreement between coders, or the reliability of coding. Any differences in coding between researchers are resolved through consensus.
This approach is more suitable for research questions that require a more structured and reliable coding process, such as in content analysis or when comparing themes across different data sets.
2. Reflexive Thematic Analysis
Braun and Clarke’s reflexive thematic analysis is an approach to qualitative data analysis that emphasizes researchers’ active role in knowledge construction.
It involves identifying patterns across data, acknowledging how researchers’ perspectives shape theme development, and critically reflecting on the analysis process throughout the study.
It acknowledges that the researcher’s subjectivity, theoretical assumptions, and interpretative framework shape the identification and interpretation of themes.
In reflexive TA, analysis starts with coding after data familiarization. Unlike other TA approaches, there is no codebook or coding frame. Instead, researchers develop codes as they work through the data.
As their understanding grows, codes can change to reflect new insights—for example, they might be renamed, combined with other codes, split into multiple codes, or have their boundaries redrawn.
If multiple researchers are involved, differences in coding are explored to enhance understanding, not to reach a consensus. The finalized coding is always open to new insights and coding.
Reflexive thematic analysis involves a more organic and iterative process of coding and theme development. The researcher continuously reflects on their role in the research process and how their own experiences and perspectives might influence the analysis.
This approach is particularly useful for exploratory research questions and when the researcher aims to provide a rich and nuanced interpretation of the data.
3. Codebook Thematic Analysis
Codebook TA, such as template, framework, and matrix analysis, combines coding reliability and reflexive elements.
Codebook TA, while employing structured coding methods like those used in coding reliability TA, generally prioritizes qualitative research values, such as reflexivity.
In this approach, the researcher develops a codebook based on their initial engagement with the data. The codebook contains a list of codes, their definitions, and examples from the data.
The codebook is then used to systematically code the entire data set. This approach allows for a more detailed and nuanced analysis of the data, as the codebook can be refined and expanded throughout the coding process.
It is particularly useful when the research aims to provide a comprehensive description of the data set.
Codebook TA is often chosen for pragmatic reasons in applied research, particularly when there are predetermined information needs, strict deadlines, and large teams with varying levels of qualitative research experience
The use of a codebook in this context helps to map the developing analysis, which is thought to improve teamwork, efficiency, and the speed of output delivery.
Why coding reliability doesn’t fit with reflexive TA:
- Using coding reliability measures in reflexive TA represents an attempt to quantify and control for subjectivity in a research approach that explicitly values the researcher’s unique contribution to knowledge construction.
- Braun and Clarke argue that such attempts to bridge the “divide” between positivist and qualitative research ultimately undermine the integrity and richness of the reflexive TA approach.
- The emphasis on coding consistency can stifle the very reflexivity that reflexive TA encourages.
Six Phases Of Reflective Thematic Analysis
Reflexive thematic analysis was developed by Virginia Braun and Victoria Clarke, two prominent qualitative researchers.
The process of thematic analysis is characterized by an iterative movement between the different phases, rather than a strict linear progression.
This means that researchers might revisit earlier phases as their understanding of the data evolves, constantly refining their analysis.
For instance, during the reviewing and developing themes phase, researchers may realize that their initial codes don’t effectively capture the nuances of the data and might need to return to the coding phase.
This back-and-forth movement continues throughout the analysis, ensuring a thorough and evolving understanding of the data.
Here’s a breakdown of the six phases:
- This initial phase involves immersing oneself in the data.
- It includes transcribing audio or video data (if necessary) and engaging in repeated readings of the transcripts.
- The goal is to gain a thorough understanding of the content and begin to notice initial patterns or interesting features.
- This phase involves systematically identifying and labeling segments of data that are relevant to the research question.
- Codes are like labels attached to meaningful chunks of data, helping to organize and categorize information.
- This phase marks the shift from individual codes to broader patterns of meaning.
- The researcher starts grouping codes that seem to cluster together, indicating potential themes.
- It’s crucial to recognize that themes do not simply “emerge” from the data; rather, the researcher actively constructs them based on their interpretation of the coded data.
- This phase involves critically evaluating the initial themes against the coded data and the entire data set.
- It’s a process of quality checking and ensuring that the themes accurately and comprehensively reflect the data.
- Researchers may need to refine, discard, or even generate new themes based on this review process.
- This phase involves developing clear and concise definitions for each theme, capturing their scope and boundaries.
- The researcher aims to identify the “essence” of each theme and ensure that each theme has a distinct and meaningful contribution to the overall analysis.
- This stage also involves developing succinct and evocative names for the themes, conveying their central meaning to the reader.
- The final phase involves weaving the themes together to present a coherent and compelling narrative of the data.
- The write-up should not merely describe the data but should offer insightful interpretations, relate the findings back to the research question, and connect them to existing literature.
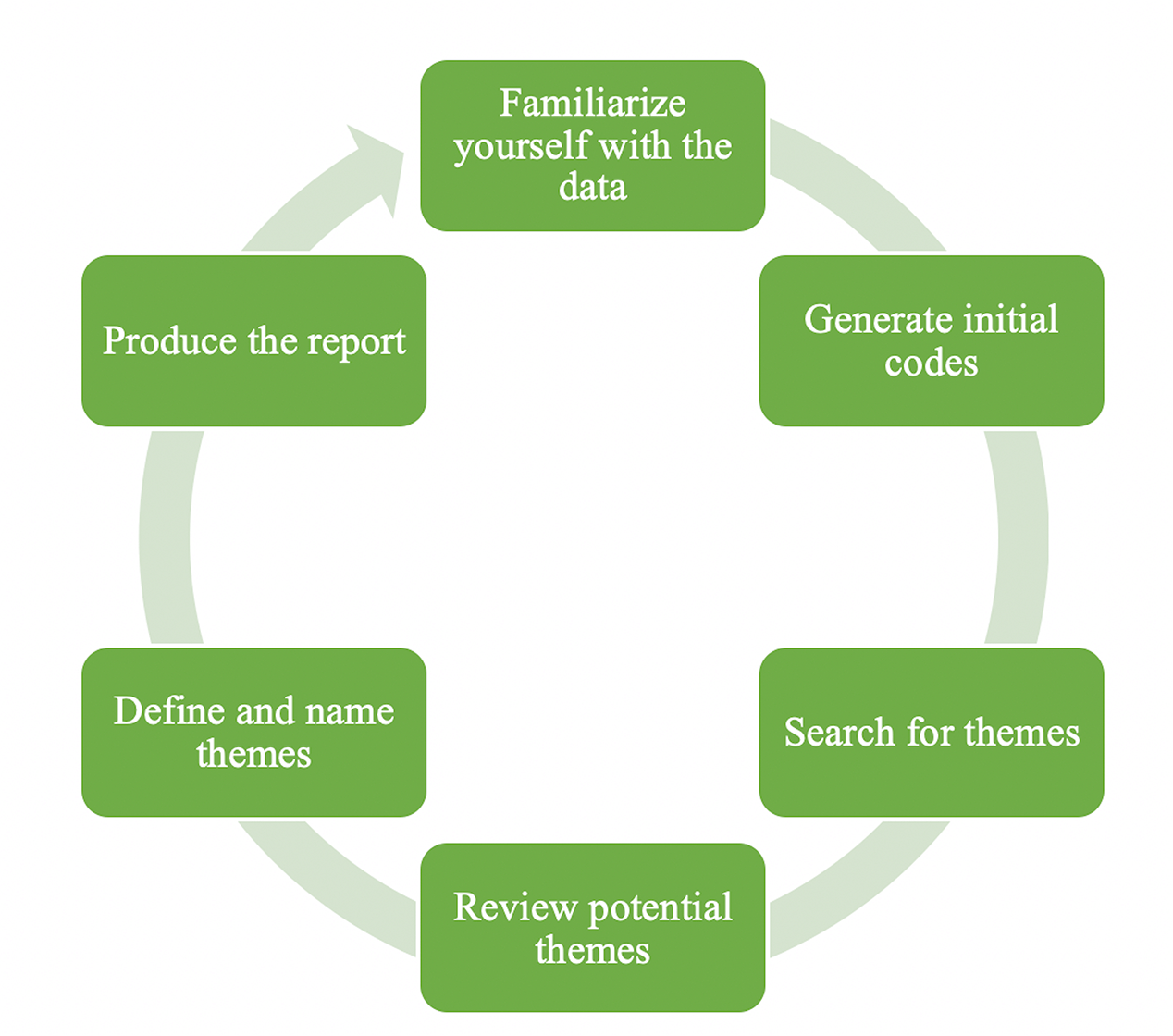
Step 1: Familiarization With the Data
Familiarization is crucial, as it helps researchers figure out the type (and number) of themes that might emerge from the data.
Familiarization involves immersing yourself in the data by reading and rereading textual data items, such as interview transcripts or survey responses.
You should read through the entire data set at least once, and possibly multiple times, until you feel intimately familiar with its content.
- Read and re-read the data (e.g., interview transcripts, survey responses, or other textual data) : The researcher reads through the entire data set multiple times to gain a comprehensive understanding of the data’s breadth and depth. This helps the researcher develop a holistic sense of the participants’ experiences, perspectives, and the overall narrative of the data.
- Listen to the audio recordings of the interviews : This helps to pick up on tone, emphasis, and emotional responses that may not be evident in the written transcripts. For instance, they might note a participant’s hesitation or excitement when discussing a particular topic. This is an important step if you didn’t collect or transcribe the data yourself.
- Take notes on initial ideas and observations : Note-making at this stage should be observational and casual, not systematic and inclusive, as you aren’t coding yet. Think of the notes as memory aids and triggers for later coding and analysis. They are primarily for you, although they might be shared with research team members.
- Immerse yourself in the data to gain a deep understanding of its content : It’s not about just absorbing surface meaning like you would with a novel, but about thinking about what the data mean .
By the end of the familiarization step, the researcher should have a good grasp of the overall content of the data, the key issues and experiences discussed by the participants, and any initial patterns or themes that emerge.
This deep engagement with the data sets the stage for the subsequent steps of thematic analysis, where the researcher will systematically code and analyze the data to identify and interpret the central themes.
Step 2: Generating Initial Codes
Codes are concise labels or descriptions assigned to segments of the data that capture a specific feature or meaning relevant to the research question.
Research question(s) and coding
- Braun and Clarke argue that the research question should be at the forefront of the researcher’s mind as they engage with the data, helping them focus their attention on what is relevant and meaningful.
- The research question is not set in stone; it can, and often should, evolve throughout the analysis.
- Braun and Clarke encourage a flexible and iterative dance between the research question and the coding process in reflexive thematic analysis.
- They advocate for a dynamic interplay where the research question guides the analysis while remaining open to refinement and even transformation based on the insights gleaned from deep engagement with the data.
- The coding process, with its close engagement with the data, can reveal new insights, nuances, and avenues for exploration, potentially leading to a reframing or narrowing of the initial research question.
The process of qualitative coding helps the researcher organize and reduce the data into manageable chunks, making it easier to identify patterns and themes relevant to the research question.
Think of it this way: If your analysis is a house, themes are the walls and roof, while codes are the individual bricks and tiles.
Coding is an iterative process, with researchers refining and revising their codes as their understanding of the data evolves.
The ultimate goal is to develop a coherent and meaningful coding scheme that captures the richness and complexity of the participants’ experiences and helps answer the research question(s).
Coding can be done manually (paper transcription and pen or highlighter) or by means of software (e.g. by using NVivo, MAXQDA or ATLAS.ti).
Qualitative data analysis software, such as NVivo can streamline the coding process, help you organize your data, and facilitate searching for patterns.
Example: Instead of manually writing codes on note cards or in separate documents, you can use software to directly tag and categorize segments of text within your data. This allows for easy retrieval and comparison of coded extracts later in the analysis
However, while software can assist with tasks like organizing codes and visually representing relationships, the researcher maintains responsibility for interpreting the data, defining themes, and making analytical decisions.
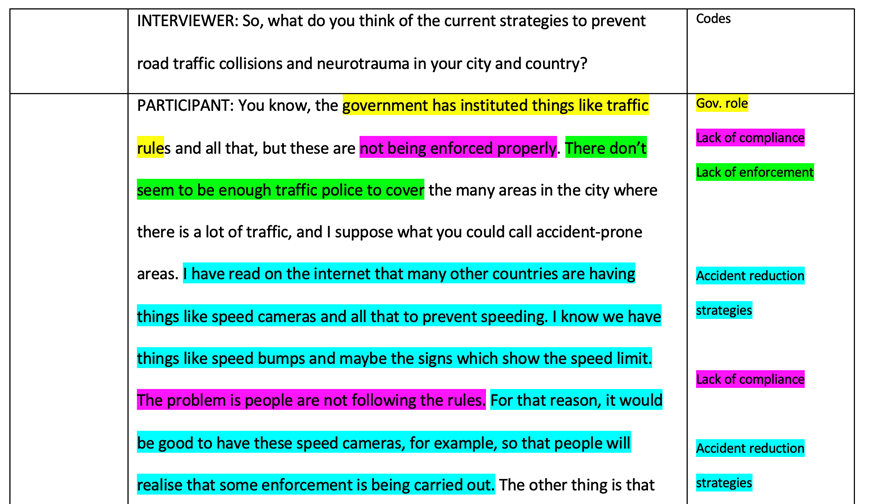
Decide On Your Coding Approach
- Will you use a predefined deductive coding framework with examples (based on theory or prior research), or let codes emerge from the data (inductive coding)?
- Will a piece of data have one code or multiple?
- Will you code everything or selectively? Broader research questions may warrant coding more comprehensively.
Instead of chasing data saturation , Clarke advocates for aiming for “ theoretical sufficiency “. This means coding data until you have enough evidence to confidently and convincingly support your interpretations and answer your research question.
If you decide not to code everything, it’s crucial to:
- Have clear criteria for what you will and won’t code.
- Be transparent about your selection process in the research report write-up.
- Remain open to revisiting uncoded data later in analysis.
Do A First Round Of Coding
- You are not required to code every single line or sentence. The size of the data segment you code can vary depending on what is meaningful and relevant to your research question.
- Go through the data and assign initial codes to chunks that could contribute to answering your research question, even if the connection seems tenuous at first.
- Instead of aiming for absolute certainty, Braun and Clarke suggest researchers consider whether a data segment is “potentially relevant” to the research question.
- Create a code name (a word or short phrase) that captures the essence of each chunk.
- Keep a codebook – a list of your codes with descriptions or definitions.
- Be open to adding, revising or combining codes as you go.
- Recognize that your understanding of the data, and therefore your codes, will likely evolve as you work through the data
After generating your first code, compare each new data extract to see if an existing code applies or if a new one is needed.
Avoid getting bogged down in trying to create the “perfect” set of codes from the outset. Embrace the iterative nature of coding, refining, and adjusting as needed
When grappling with the decision of whether to code a particular data segment, Braun and Clarke advocate for an inclusive approach, particularly in the initial stages of analysis.
They emphasize that it’s easier to discard codes later than to revisit the entire dataset for recording.
Coding can be done at two levels of meaning:
Semantic codes provide a descriptive snapshot of the data, while latent codes offer a more interpretive and deeper understanding of the underlying meanings and assumptions present.
- Semantic: These codes capture the surface meaning or explicit content of the data. They stay close to the participants’ intended meaning, mirroring their language and concepts. Think of semantic codes as a direct representation of what the participant says, with minimal interpretation by the researcher. They provide a concise summary of a portion of data, staying close to the content and the participant’s meaning.
- Latent: Goes beyond the participant’s meaning to provide a conceptual interpretation of the data. They often draw on existing theories or concepts to interpret the data, providing a more conceptual “take” on what the participants are saying. Latent codes require the researcher to dig beneath the surface and make inferences based on their expertise and knowledge.
The decision of whether to use semantic or latent codes, or a mix of both, depends on the research question, the specific data, and the theoretical orientation of the researcher.
Latent coding requires more experience and theoretical knowledge than semantic coding.
Most codes will be a mix of descriptive and conceptual. Novice coders tend to generate more descriptive codes initially, developing more conceptual approaches with experience.
Both types of codes are valuable in thematic analysis and contribute to a more comprehensive and insightful analysis of qualitative data.
Evolution of codes:
Coding in reflexive TA is not a linear, pre-determined process; instead, it’s an iterative process characterized by constant development, refinement, and transformation.
Braun and Clarke underscore that in reflexive TA, codes are not static categories but rather evolving tools that the researcher actively shapes and reshapes in response to the emerging insights from the data.
Don’t be afraid to revisit and adjust your codes —this is a sign of thoughtful engagement, not failure.
Braun and Clark highlight how codes might be:
- Renamed: As the researcher’s understanding of the data deepens, they might find that a code’s initial label no longer accurately reflects the nuances of the meaning it captures. Renaming allows for a more precise and insightful representation of the data.
- Combined: Codes that initially seemed distinct might reveal overlaps or shared connections as the analysis progresses, leading to their merging into a broader, more encompassing code.
- Split: Conversely, a code that initially seemed cohesive might later reveal subtle distinctions within it, prompting the researcher to split it into two or more more focused codes, reflecting a more nuanced understanding of the data.
- Redrawn boundaries: The scope and focus of a code can also shift throughout the analysis, leading to a redrawing of its boundaries to better encapsulate the emerging patterns and insights.
This step ends when:
- All data is fully coded.
- Data relevant to each code has been collated.
You have enough codes to capture the data’s diversity and patterns of meaning, with most codes appearing across multiple data items.
The number of codes you generate will depend on your topic, data set, and coding precision.
Step 3: Generating Initial Themes
Generating initial provisional (candidate) themes begins after all data has been initially coded and collated, resulting in a comprehensive list of codes identified across the data set.
This step involves shifting from the specific, granular codes to a broader, more conceptual level of analysis.
What is the difference between a theme and a code?
- A code is attached to a segment of data (your “coding chunk”) that is potentially relevant to your research question
- Themes are built from codes, meaning they’re more abstract and interpretive.
- Codes capture a single idea or observation, while a theme pulls together multiple codes to create a broader, more nuanced understanding of the data.
- Think of codes as the building blocks, and themes as the structure you create using those blocks.
Themes are higher-level units of analysis that organize and interpret the codes, revealing the overarching stories and key insights within the data. The focus is on making sense of the coded data by identifying connections, similarities, and overarching patterns that address the research question.
Phase 3 of thematic analysis is about actively “generating initial themes” rather than passively “searching for themes.” The distinction highlights that researchers don’t just uncover pre-existing themes hidden within the data.
Thematic analysis is not about “discovering” themes that already exist in the data, but rather actively constructing or generating themes through a careful and iterative process of examination and interpretation.
Themes involve a higher level of abstraction and interpretation. They go beyond merely summarizing the data (what participants said) and require the researcher to synthesize codes into meaningful clusters that offer insights into the underlying meaning and significance of the findings in relation to the research question.
Collating codes into potential themes :
The generating initial themes step helps the researcher move from a granular, code-level analysis to a more conceptual, theme-level understanding of the data.
The process of collating codes into potential themes involves grouping codes that share a unifying feature or represent a coherent and meaningful pattern in the data.
The researcher looks for patterns, similarities, and connections among the codes to develop overarching themes that capture the essence of the data.
It’s important to remember that coding is an organic and ongoing process.
You may need to re-read your entire data set to see if you have missed any data relevant to your themes, or if you need to create any new codes or themes.
Once a potential theme is identified, all coded data extracts associated with the codes grouped under that theme are collated. This ensures a comprehensive view of the data pertaining to each theme.
The researcher should ensure that the data extracts within each theme are coherent and meaningful.
This step helps ensure that your themes accurately reflect the data and are not based on your own preconceptions.
By the end of this step, the researcher will have a collection of candidate themes (and maybe sub-themes), along with their associated data extracts.
However, these themes are still provisional and will be refined in the next step of reviewing the themes.
This process is similar to sculpting, where the researcher shapes the “raw” data into a meaningful analysis. This involves grouping codes that share a unifying feature or represent a coherent pattern in the data:
- Review the list of initial codes and their associated data extracts (e.g., highlighted quotes or segments from interview transcripts).
- Look for codes that seem to share a common idea or concept.
- Group related codes together to form potential themes.
- If using qualitative data analysis software, you can assign the coded extracts to the relevant themes within the software.
- Some codes may form main themes, while others may be sub-themes or may not fit into any theme.
- If a coded extract seems to fit under multiple themes, choose the theme that it most closely aligns with in terms of shared meaning.
Example : The researcher would gather all the data extracts related to “Financial Obstacles and Support,” such as quotes about struggling to pay for tuition, working long hours, or receiving scholarships.
Thematic maps
Thematic maps can help visualize the relationship between codes and themes. These visual aids provide a structured representation of the emerging patterns and connections within the data, aiding in understanding the significance of each theme and its contribution to the overall research question.
- As you identify which theme each coded extract belongs to, copy and paste the extract under the relevant theme in your thematic map or table.
- Include enough context around each extract to ensure its meaning is clear.
Thematic maps often use visual elements like boxes, circles, arrows, and lines to represent different codes and themes and to illustrate how they connect to one another.
Thematic maps typically display themes and subthemes in a hierarchical structure, moving from broader, overarching themes to more specific, nuanced subthemes.
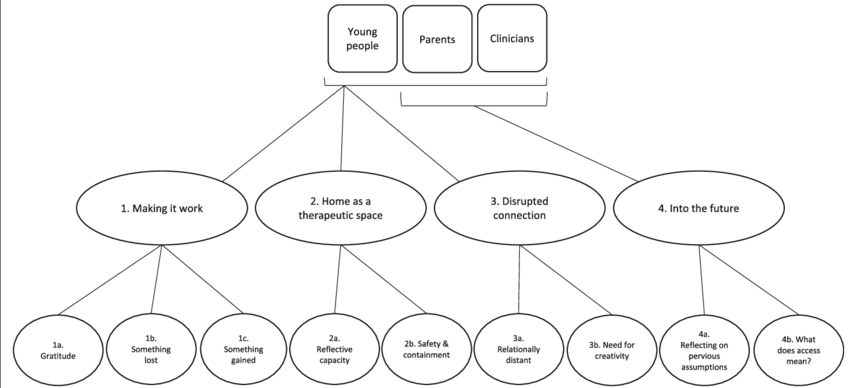
Maps can help researchers visualize the connections and tensions between different themes, revealing how they intersect or diverge to create a more nuanced understanding of the data.
Similar to the iterative nature of thematic analysis itself, thematic maps are fluid and adaptable, changing as the researcher gains a deeper understanding of the data.
Maps can highlight overlaps between themes or areas where a theme might be too broad or too narrow, prompting the researcher to adjust their analysis accordingly.
Example : Studying first-generation college students, the researcher might notice that the codes “financial challenges,” “working part-time,” and “scholarships” all relate to the broader theme of “Financial Obstacles and Support.”
Two main conceptualizations of a theme exist:
- Bucket theme (domain summary) : This approach identifies a pre-defined area of interest (often from interview questions) and summarizes all data relevant to that area.
- Storybook theme (shared meaning) : This approach focuses on identifying broader patterns of meaning that tell a story about the data. These themes go beyond simply summarizing and involve a greater degree of interpretation from the researcher.
Avoid : Themes as Domain Summaries (Shared Topic or “Bucket Themes”)
Domain summary themes are organized around a shared topic but not a shared meaning, and often resemble “buckets” into which data is sorted.
A domain summary organizes data around a shared topic but not a shared meaning.
In this approach, themes simply summarize what participants mentioned about a particular topic, without necessarily revealing a unified meaning.
Domain summaries group data extracts around a common topic or area of inquiry, often reflecting the interview questions or predetermined categories.
The emphasis is on collating all relevant data points related to that topic, regardless of whether they share a unifying meaning or concept.
While potentially useful for organizing data, domain summaries often remain at a descriptive level, failing to offer deeper insights into the data’s underlying meanings and implications.
These themes are often underdeveloped and lack a central organizing concept that ties all the different observations together.
A strong theme has a “central organizing concept” that connects all the observations and interpretations within that theme and goes beyond surface-level observations to uncover implicit meanings and assumptions.
A theme should not just be a collection of unrelated observations of a topic. This means going beyond just describing the “surface” of the data and identifying the assumptions, conceptualizations, and ideologies that inform the data’s meaning.
It’s crucial to avoid creating themes that are merely summaries of data domains or directly reflect the interview questions.
Example 1 : A theme titled “Incidents of homophobia” that merely describes various participant responses about homophobia without delving into deeper interpretations would be a topic summary theme.
Example 2 : A theme titled “Benefits of Being Single” that lists all the positive aspects of singlehood mentioned by participants would be a domain summary. A more insightful theme might explore the underlying reasons behind these benefits, such as “Redefining Independence in Singlehood.”
Tip : Using interview questions as theme titles without further interpretation or relying on generic social functions (“social conflict”) or structural elements (“economics”) as themes often indicates a lack of shared meaning and thorough theme development. Such themes might lack a clear connection to the specific dataset
Ensure : Themes as Shared Meaning (or “Storybook Themes”)
Braun and Clarke stress that a theme should offer more than a mere description of the data; it should tell a story about the data.
Instead, themes should represent a deeper level of interpretation, capturing the essence of the data and providing meaningful insights into the research question.
Shared meaning themes are patterns of shared meaning underpinned by a central organizing concept.
In contrast to domain summaries, shared meaning themes go beyond merely identifying a topic. They are organized around a “ central organizing concept ” that ties together all the observations and interpretations within that theme.
This central organizing concept represents the researcher’s interpretation of the shared meaning that connects seemingly disparate data points.
They reflect a pattern of shared meaning across different data points, even if those points come from different topics.
- Emphasis on interpretation and insight: Shared meaning themes require the researcher to move beyond surface-level descriptions and engage in a more interpretive and nuanced analysis. This involves identifying the underlying assumptions, conceptualizations, and ideologies that shape participants’ experiences and perspectives.
- Themes as interpretive stories: Braun and Clarke use the metaphor of a “storybook” to capture the essence of shared meaning themes. These themes aim to tell a compelling and insightful story about the data, going beyond a mere restatement of what participants said.
Example : The theme “‘There’s always that level of uncertainty’: Compulsory heterosexuality at university” effectively captures the shared experience of fear and uncertainty among LGBT students, connecting various codes related to homophobia and its impact on their lives.
Key considerations for developing shared meaning themes:
- Identifying the “Essence”: Developing a strong shared meaning theme involves identifying the “essence” or “core idea” that underpins a cluster of codes and data extracts. This requires asking questions like: What is the common thread that connects these observations? What underlying assumptions or beliefs are being expressed? What is the larger story that these data points tell about the phenomenon being studied?
- Moving beyond the literal: Shared meaning themes often involve uncovering the implicit or latent meanings embedded within the data. This requires the researcher to look beyond the literal interpretations of participants’ words and consider the broader social and cultural contexts that shape their perspectives.
Step 4: Reviewing Themes
The researcher reviews, modifies, and develops the preliminary themes identified in the previous step, transforming them into final, well-developed themes.
This phase involves a recursive process of checking the themes against the coded data extracts and the entire data set to ensure they accurately reflect the meanings evident in the data.
The purpose is to refine the themes, ensuring they are coherent, consistent, and distinctive.
According to Braun and Clarke, a well-developed theme “captures something important about the data in relation to the research question and represents some level of patterned response or meaning within the data set”.
A well-developed theme will:
- Go beyond paraphrasing the data to analyze the meaning and significance of the patterns identified.
- Provide a detailed analysis of what the theme is about.
- Be supported with a good amount of relevant data extracts.
- Be related to the research question.
Revisions at this stage might involve creating new themes, refining existing themes, or discarding themes that do not fit the data. For example, you might realize that two provisional themes actually overlap significantly and decide to merge them into a single, more nuanced theme.
Level One : Reviewing Themes Against Coded Data Extracts
- Researchers begin by comparing their initial candidate themes against the coded data extracts associated with each theme to ensure they form a coherent pattern.
- This step helps to determine whether each theme is supported by the data and whether it accurately reflects the meaning found in the extracts. Determine if there is enough data to support each theme.
- Look at the relationships between themes and sub-themes in the thematic map. Consider whether the themes work together to tell a coherent story about the data. If the thematic map does not effectively represent the data, consider making adjustments to the themes or their organization.
- If some extracts do not fit well with the rest of the data in a theme, consider whether they might better fit under a different theme or if the theme needs to be refined.
- It’s important to ensure that each theme has a singular focus and is not trying to encompass too much. Themes should be distinct from one another, although they may build on or relate to each other.
- Discarding codes : If certain codes within a theme are not well-supported or do not fit, they can be removed.
- Relocating codes : Codes that fit better under a different theme can be moved.
- Redrawing theme boundaries : The scope of a theme can be adjusted to better capture the relevant data.
- Discarding themes : Entire themes can be abandoned if they do not work.
Level Two : Evaluating Themes Against the Entire Data Set
- Once the themes appear coherent and well-supported by the coded extracts, researchers move on to evaluate them against the entire data set.
- This involves a final review of all the data to ensure that the themes accurately capture the most important and relevant patterns across the entire dataset in relation to the research question.
- During this level, researchers may need to recode some extracts for consistency, especially if the coding process evolved significantly, and earlier data items were not recoded according to these changes.
Level Three : Considering relationships between codes, themes, and different levels of themes (sub-themes)
Once you have gathered all the relevant data extracts under each theme, review the themes to ensure they are meaningful and distinct.
This step involves analyzing how different codes combine to form overarching themes and exploring the hierarchical relationship between themes and sub-themes.
Within a theme, there can be different levels of themes, often organized hierarchically as main themes and sub-themes.
Some themes may be more prominent or overarching (main themes), while others may be secondary or subsidiary (sub-themes).
- Main themes represent the most overarching or significant patterns found in the data. They provide a high-level understanding of the key issues or concepts present in the data.
- Sub-themes are essentially themes within a theme. They represent a further level of nuance and complexity within a broader theme, highlighting specific and important aspects of the central organizing concept of that theme.
Sub-themes provide a way to add depth and richness to your thematic analysis, but they should be used thoughtfully and strategically. A well-structured analysis might rely primarily on clearly defined main themes, using sub-themes selectively to highlight particularly important nuances within those themes.
Too many sub-themes can create a thin, fragmented analysis and suggest that the analysis hasn’t been developed sufficiently to identify the overarching concepts that tie the data together.
It’s important to note that sub-themes are not a necessary feature of a reflexive TA. You can have a robust analysis with just two to six main themes, especially if you are working with a limited word count
The relationship between codes, sub-themes and main themes can be visualized using a thematic map, diagram, or table.
This map helps researchers review and refine themes, ensuring they are internally consistent (homogeneous) and distinct from other themes (heterogeneous).
Refine the thematic map as you continue to review and analyze the data.
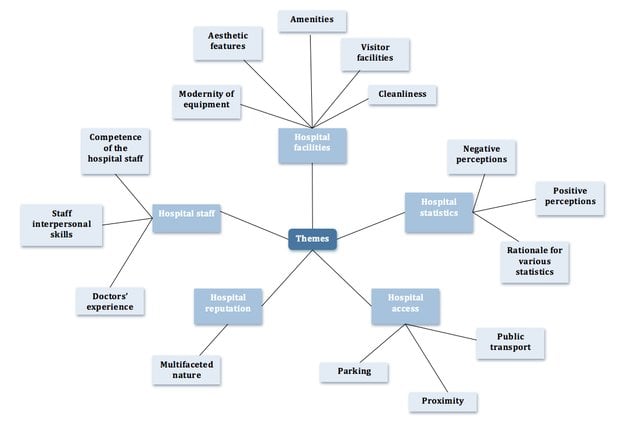
Consider how the themes tell a coherent story about the data and address the research question.
If some themes seem to overlap or are not well-supported by the data, consider combining or refining them.
If a theme is too broad or diverse, consider splitting it into separate themes or sub-theme.
Example : The researcher might identify “Academic Challenges” and “Social Adjustment” as other main themes, with sub-themes like “Imposter Syndrome” and “Balancing Work and School” under “Academic Challenges.” They would then consider how these themes relate to each other and contribute to the overall understanding of first-generation college students’ experiences.
Final Questions:
- Does this provisional theme capture something meaningful? Is it coherent, with a central idea that meshes the data and codes together? Does it have clear boundaries?”
- “Can I identify the boundaries of this theme?”
- “Are there enough meaningful data to evidence this theme?”
- “Are there multiple articulations around the core idea, and are they nuanced, complex, and diverse?”
- “Does the theme feel rich?”
- “Are the data contained within each theme too diverse and wide-ranging?”
- “Does the theme convey something important?”
Step 5: Defining and Naming Themes
The themes are finalized when the researcher is satisfied with the theme names and definitions.
If the analysis is carried out by a single researcher, it is recommended to seek feedback from an external expert to confirm that the themes are well-developed, clear, distinct, and capture all the relevant data.
Defining themes means determining the exact meaning of each theme and understanding how it contributes to understanding the data.
This process involves formulating exactly what we mean by each theme. The researcher should consider what a theme says, if there are subthemes, how they interact and relate to the main theme, and how the themes relate to each other.
Themes should not be overly broad or try to encompass too much, and should have a singular focus. They should be distinct from one another and not repetitive, although they may build on one another.
In this phase the researcher specifies the essence of each theme.
- What does the theme tell us that is relevant for the research question?
- How does it fit into the ‘overall story’ the researcher wants to tell about the data?
Naming themes involves developing a clear and concise name that effectively conveys the essence of each theme to the reader. A good name for a theme is informative, concise, and catchy.
- A well-crafted theme name should immediately convey the theme’s central organizing concept and give the reader a sense of the story the theme will tell.
- The researcher develops concise, punchy, and informative names for each theme that effectively communicate its essence to the reader.
- Theme names should be catchy and evocative, giving the reader an immediate sense of what the theme is about.
- Avoid using one-word theme names or names that simply identify the topic, as this often signifies a domain summary rather than a well-developed theme.
- Avoid using jargon or overly complex language in theme names.
- The name should go beyond simply paraphrasing the content of the data extracts and instead interpret the meaning and significance of the patterns within the theme.
- The goal is to make the themes accessible and easily understandable to the intended audience. If a theme contains sub-themes, the researcher should also develop clear and informative names for each sub-theme.
- Theme names can include direct quotations from the data, which helps convey the theme’s meaning. However, researchers should avoid using data collection questions as theme names. Using data collection questions as themes often leads to analyses that present domain summaries of topics rather than fully realized themes.
For example, “‘There’s always that level of uncertainty’: Compulsory heterosexuality at university” is a strong theme name because it captures the theme’s meaning. In contrast, “incidents of homophobia” is a weak theme name because it only states the topic.
For instance, a theme labeled “distrust of experts” might be renamed “distrust of authority” or “conspiracy thinking” after careful consideration of the theme’s meaning and scope.
Step 6: Producing the Report
Braun and Clarke differentiate between two distinct approaches to presenting the analysis in qualitative research: the “establishing the gap model” and the “making the argument model” (p.120).
Establishing the Gap Model:
This model operates on the premise that knowledge gaps exist due to limited research in specific areas or shortcomings in current research.
This approach frames the research’s purpose as filling these identified gaps. Braun and Clarke critique this model as echoing a positivist-empiricist view of research as a quest for definitive truth, which they argue is incongruent with the nature of qualitative research.
They suggest this approach aligns more with a quantitative perspective that seeks to uncover objective truths.
Making the Argument Model:
Braun and Clarke advocate for the “making the argument model,” particularly in the context of qualitative research.
This model situates the research’s rationale within existing knowledge and theoretical frameworks.
Rather than striving to unearth a singular truth, this approach aims to contribute to a comprehensive and nuanced understanding of the subject matter by offering a well-supported, contextually grounded, and persuasive perspective on the issue at hand.
This approach might negate the need for a literature review before data analysis, allowing the research findings to guide the exploration of relevant literature.
Method Section of Thematic Analysis
A well-crafted method section goes beyond a superficial summary of the six phases.
It provides a clear and comprehensive account of the analytical journey, allowing readers to trace the researchers’ thought process, assess the trustworthiness of the findings, and understand the rationale behind the methodological choices made.
This transparency is essential for ensuring the rigor and validity of thematic analysis as a qualitative research method.
1. Description of the thematic approach:
The method section should explicitly state the type of thematic analysis undertaken and the specific version used (e.g., reflexive thematic analysis, codebook thematic analysis).
It should also explain the rationale for selecting this specific approach in relation to the research questions.
For instance, if a study focuses on exploring participants’ lived experiences, an inductive (reflexive) approach might be more suitable.
If the research question is driven by a particular theoretical framework, a deductive (codebook) approach may be chosen.
2. Data collection method and data set:
Clearly describe the method used to collect data (e.g., interviews, focus groups , surveys, documents).
Specify the size of the data set (e.g., number of interviews, focus groups, or documents) and the characteristics of the participants or texts included.
3. Reflexivity and transparency:
Braun and Clarke caution against merely listing the six phases of thematic analysis because presenting the phases as a series of steps implies that thematic analysis is a linear and objective process that can be separated from the researcher’s influence.
It should demonstrate an understanding of the principles of reflexivity and transparency.
By embracing reflexivity and transparency, researchers using thematic analysis can move away from a simplistic “recipe” approach and acknowledge the iterative and interpretive nature of qualitative research.
Reflexivity involves acknowledging and critically examining how the researcher’s own subjectivity might be shaping the research process.
It requires reflecting on how personal experiences, beliefs, and assumptions could influence the interpretation of data and the development of themes.
For example, a researcher studying experiences of discrimination might reflect on how their own social identities and experiences with prejudice could impact their understanding of the data.
Transparency involves clearly documenting the decisions made throughout the research process.
This includes explaining the rationale behind coding choices, theme development, and the selection of data extracts to illustrate themes.
For example, the researcher(s) might discuss the process of selecting particular data extracts or how their initial interpretations evolved over time.
Transparency allows readers to understand how the findings were generated and to assess the trustworthiness of the research.
The researcher(s) could provide a detailed account of how they moved from initial codes to broader themes, including examples of how they resolved discrepancies between codes or combined them into overarching categories.
While transparency requires detail and rigor, it should not come at the expense of clarity and accessibility.
Braun and Clarke encourage researchers to write in a clear, engaging style that makes the research process and findings accessible to a wide audience, including those who might not be familiar with qualitative research methods.
Writing About Themes
A thematic analysis report should provide a convincing and clear, yet complex story about the data that is situated within a scholarly field.
A balance should be struck between the narrative and the data presented, ensuring that the report convincingly explains the meaning of the data, not just summarizes it.
To achieve this, the report should include vivid, compelling data extracts illustrating the themes and incorporate extracts from different data sources to demonstrate the themes’ prevalence and strengthen the analysis by representing various perspectives within the data.
The report should be written in first-person active tense, unless otherwise stated in the reporting requirements.
The analysis can be presented in two ways :
- Integrated Results and Discussion section: This approach is suitable when the analysis has strong connections to existing research and when the analysis is more theoretical or interpretive.
- Separate Discussion section: This approach presents the data interpretation separately from the results.
Regardless of the presentation style, researchers should aim to “show” what the data reveals and “tell” the reader what it means in order to create a convincing analysis.
- Presentation order of themes: Consider how to best structure the presentation of the themes in the report. This may involve presenting the themes in order of importance, chronologically, or in a way that tells a coherent story. The order in which themes are presented should be logical and meaningful, creating a clear storyline for the reader.
- Subheadings: Use subheadings to clearly delineate each theme and its sub-themes, making the report easy to navigate and understand.
Themes should connect logically and meaningfully and, if relevant, should build on previous themes to tell a coherent story about the data.
Avoid using phrases like “themes emerged” as it suggests that the themes were pre-existing entities in the data, waiting to be discovered. This undermines the active role of the researcher in interpreting and constructing themes from the data.
Themes should be supported with compelling data extracts that illustrate the identified patterns.
Data extracts serve as evidence for the themes identified in TA. Without them, the analysis becomes unsubstantiated and potentially unconvincing to the reader.
The report should include vivid, compelling data extracts that clearly illustrate the theme being discussed and should incorporate extracts from different data sources, rather than relying on a single source.
Not all data extracts are equally effective. Choose extracts that vividly and concisely illustrate the theme’s central organizing concept.
Although it is tempting to rely on one source when it eloquently expresses a particular aspect of the theme, using multiple sources strengthens the analysis by representing a wider range of perspectives within the data.
Having too few data extracts for a theme weakens the analysis and makes it appear “thin and sketchy”. This may leave the reader unconvinced about the theme’s validity and prevalence within the data.
The analysis should go beyond a simple summary of the participant’s words and instead interpret the meaning of the data.
Data extracts should not be presented without being integrated into the analytic narrative. They should be used to illustrate and support the interpretation of the data, not just reiterate what the participants said.
Researchers should strive to maintain a balance between the amount of narrative and the amount of data presented.
A good thematic analysis strikes a balance between presenting data extracts and providing analytic commentary. A common rule of thumb is to aim for a 50/50 ratio.
The importance of examining contradictory data
A robust thematic analysis acknowledges and explores the full range of data, including those that challenge the dominant patterns.
Ignoring data that doesn’t neatly fit into identified themes is a significant pitfall in thematic analysis.
Failing to acknowledge and explore contradictory data can lead to an incomplete or misleading analysis, potentially obscuring valuable insights.
- Data sets are rarely completely uniform : Human experiences and perspectives are complex and often contradictory. It’s unrealistic to expect that every piece of data will perfectly align with the identified themes.
- Contradictory data can challenge assumptions : Data that contradicts the emerging themes can challenge the researcher’s assumptions and interpretations, leading to a more nuanced and insightful understanding of the data.
- Ignoring contradictions can create an overly simplistic analysis : An analysis that smooths over contradictions or presents a completely unified picture of the data might lack depth and fail to capture the complexities of the phenomenon being studied.
- Alternative interpretations : Contradictory data might suggest alternative interpretations or explanations that need to be considered and addressed in the analysis.
- Value of outliers : Instead of dismissing data that doesn’t fit, view it as potentially valuable. These outliers might reveal limitations in the analysis, highlight the influence of contextual factors, or uncover new avenues for inquiry.
Embracing contradictions and exploring their potential meanings leads to a more comprehensive and insightful analysis.
Discussion Section
The discussion section should engage critically with the findings, connect them to existing knowledge, and contribute to a deeper understanding of the phenomenon under investigation.
Braun and Clarke emphasize that the discussion section should not merely summarize the themes but rather weave a compelling and insightful narrative that connects the analysis back to the research question, existing literature, and broader theoretical discussions.
While each theme should have a distinct focus, the discussion should also draw connections between themes, creating a cohesive and interconnected narrative.
They advocate for a style that engages the reader, convinces them of the validity of the findings, and leaves them with a sense of “ so what? ” – a clear understanding of the significance and implications of the research.
- Connecting themes and building a narrative: The discussion section should move beyond simply describing individual themes to explore the relationships and connections between them. The goal is to present a coherent and nuanced narrative that addresses the research questions.
- Interpreting the findings: The discussion section should interpret the significance of the findings about the research questions and existing literature. It should go beyond merely summarizing the data to offer insights into what the themes mean, why they might have emerged, and what their implications are. Asking questions like “So what?” and “What is relevant or useful here to addressing my question?” can help you guide the interpretation of the data.
- Integrating literature: The discussion section should connect the findings to relevant scholarly literature. This could involve comparing and contrasting the findings with previous research, exploring how the study supports or challenges existing theories, or discussing the implications of the findings in light of existing knowledge.
- Theoretical insights: For analyses that go beyond the semantic level, the discussion section should explore the theoretical insights that emerge from the data. This could involve identifying underlying assumptions, ideologies, or power dynamics that shape the experiences or perspectives of the participants.
- Critical reflection on the method: Reflect on the methodological choices made during the analysis and their potential implications for the findings. This could involve discussing the benefits and limitations of the chosen thematic analysis approach, acknowledging any potential biases, and suggesting areas for future research.
Potential Pitfalls to Avoid
- Failing to analyze the data : Thematic analysis should involve more than simply presenting data extracts without an analytic narrative. The researcher must provide an interpretation and make sense of the data, telling the reader what it means and how it relates to the research questions.
- Using data collection questions as themes : Themes should be identified across the entire dataset, not just based on the questions asked during data collection. Reporting data collection questions as themes indicates a lack of thorough analytic work to identify patterns and meanings in the data.
- Confusing themes with summaries : Themes are not merely summaries of what participants said about a topic. Instead, they represent rich and multifaceted patterns of shared meaning organized around a central concept and are generated by the researcher through intense analytic engagement with the data. Good themes often uncover the implicit or latent meanings behind the data rather than just summarizing what’s explicitly stated.
- Conducting a weak or unconvincing analysis : Themes should be distinct, internally coherent, and consistent, capturing the majority of the data or providing a rich description of specific aspects. A weak analysis may have overlapping themes, fail to capture the data adequately, or lack sufficient examples to support the claims made.
- Ignoring contradictory data : An analysis that smooths over contradictions or presents a completely unified picture of the data might lack depth and fail to capture the complexities of the phenomenon being studied. Acknowledging and exploring data that does not fit neatly into identified themes can lead to more nuanced findings.
- Mismatch between data and analytic claims : The researcher’s interpretations and analytic points must be consistent with the data extracts presented. Claims that are not supported by the data, contradict the data, or fail to consider alternative readings or variations in the account are problematic.
- Misalignment between theory, research questions, and analysis : The interpretations of the data should be consistent with the theoretical framework used. For example, an experiential framework would not typically make claims about the social construction of the topic. The form of thematic analysis used should also align with the research questions.
- Neglecting to clarify assumptions, purpose, and process : A good thematic analysis should spell out its theoretical assumptions, clarify how it was undertaken, and for what purpose. Without this crucial information, the analysis is lacking context and transparency, making it difficult for readers to evaluate the research.
Reducing Bias
Braun and Clarke’s approach to thematic analysis, which they term “reflexive TA,” places the researcher’s subjectivity and reflexivity at the forefront of the research process.
Rather than striving for an illusory objectivity, reflexive TA recognizes and values the researcher’s active role in shaping the research, from data interpretation to theme construction.
When researchers are both reflexive and transparent in their thematic analysis, it strengthens the trustworthiness and rigor of their findings.
The explicit acknowledgement of potential biases and the detailed documentation of the analytical process provide a stronger foundation for the interpretation of the data, making it more likely that the findings reflect the perspectives of the participants rather than the biases of the researcher.
Reflexivity
Reflexivity involves critically examining one’s own assumptions and biases, is crucial in qualitative research to ensure the trustworthiness of findings.
It requires acknowledging that researcher subjectivity is inherent in the research process and can influence how data is collected, analyzed, and interpreted.

Identifying and Challenging Assumptions:
Braun and Clarke argue that the researcher’s background, experiences, theoretical commitments, and social position inevitably shape how they approach and make sense of the data.
Reflexivity encourages researchers to explicitly acknowledge their preconceived notions, theoretical leanings, and potential biases.
Reflexivity involves critically examining how these personal and professional experiences influence the research process, particularly during data interpretation and theme development.
Researchers are encouraged to make these influences transparent in their methodology and throughout their analysis, fostering a more honest and nuanced account of the research.
Memos offer a space for researchers to step back from the data and ask themselves probing questions about their own perspectives and potential biases.
Researchers can ask: How might my background or beliefs be shaping my interpretation of this data? Am I overlooking alternative explanations? Am I imposing my own values or expectations on the participants?
By actively reflecting on how these factors might influence their interpretation of the data, researchers can take steps to mitigate their impact.
This might involve seeking alternative explanations, considering contradictory evidence, or discussing their interpretations with others to gain different perspectives.
Reflexivity as an Ongoing Process
Reflexivity is not a one-time activity but an ongoing process that should permeate all stages of the research, from the initial design to the final write-up.
This involves constantly questioning one’s assumptions, interpretations, and reactions to the data, considering alternative perspectives, and remaining open to revising initial understandings.
Braun and Clarke provide a series of probing questions that researchers can ask themselves throughout the analytic process to encourage this reflexivity.
- “Why might I be reacting to the data in this way?”
- “What does my interpretation rely on?”
- “How would I feel if I was in that situation? (Is this different from or similar to how the person feels, and why might that be?)”
Transparency
Transparency refers to clearly documenting the research process, including coding decisions, theme development, and the rationale behind behind theme development.
Transparency is not merely about documenting what was done but also about clearly articulating why and how specific analytic choices were made throughout the research process, from study design to data interpretation.
This transparency allows readers to understand the researchers’ perspectives, the rationale behind their decisions, and the potential influences on the findings, ultimately strengthening the credibility and trustworthiness of the research
This transparency helps ensure the trustworthiness and rigor of the findings, allowing other researchers to assess the credibility of the findings and potentially replicate the analysis.
Transparency in Braun and Clarke’s approach to thematic analysis is not merely about adhering to a set of reporting guidelines; it’s about embracing an ethos of openness, reflexivity, and accountability throughout the research process.
By illuminating the “messiness” of qualitative research and clearly articulating the researchers’ perspectives and decisions, reflexive TA promotes a more honest, trustworthy, and ultimately, more insightful form of qualitative inquiry.
Documenting Decision-Making:
Transparency requires researchers to provide a clear and detailed account of their analytical choices throughout the research process.
This includes documenting the rationale behind coding decisions, the process of theme development, and any changes made to the analytical approach during the study.
- Data selection and sampling: Why were particular data sources chosen? How were participants selected, and what were the inclusion/exclusion criteria?
- Coding strategies: How were codes developed? Was the coding primarily inductive, deductive, or a combination of both? Did the coding process evolve, and if so, how? Were any coding tools or software used?
- Theme development: How were themes identified, refined, and named? What was the process of moving from codes to themes? How was the final thematic structure decided upon?
By making these decisions transparent, researchers allow others to scrutinize their work and assess the potential for bias.
Practical Strategies for Reflexivity and Transparency in Thematic Analysis:
- Maintaining a reflexive journal: Researchers can keep a journal throughout the research process to document their thoughts, assumptions, and potential biases. This journal serves as a record of the researcher’s evolving understanding of the data and can help identify potential blind spots in their analysis.
- Engaging in team-based analysis: Collaborative analysis, involving multiple researchers, can enhance reflexivity by providing different perspectives and interpretations of the data. Discussing coding decisions and theme development as a team allows researchers to challenge each other’s assumptions and ensure a more comprehensive analysis.
- Clearly articulating the analytical process: In reporting the findings of thematic analysis, researchers should provide a detailed account of their methods, including the rationale behind coding decisions, the process of theme development, and any challenges encountered during analysis. This transparency allows readers to understand the steps taken to ensure the rigor and trustworthiness of the analysis.
- Flexibility: Thematic analysis is a flexible method, making it adaptable to different research questions and theoretical frameworks. It can be employed with various epistemological approaches, including realist, constructionist, and contextualist perspectives. For example, researchers can focus on analyzing meaning across the entire data set or examine a particular aspect in depth.
- Accessibility: Thematic analysis is an accessible method, especially for novice qualitative researchers, as it doesn’t demand extensive theoretical or technical knowledge compared to methods like Discourse Analysis (DA) or Conversation Analysis (CA). It is considered a foundational qualitative analysis method.
- Rich Description: Thematic analysis facilitates a rich and detailed description of data9. It can provide a thorough understanding of the predominant themes in a data set, offering valuable insights, particularly in under-researched areas.
- Theoretical Freedom: Thematic analysis is not restricted to any pre-existing theoretical framework, allowing for diverse applications. This distinguishes it from methods like Grounded Theory or Interpretative Phenomenological Analysis (IPA), which are more closely tied to specific theoretical approaches
Disadvantages
- Subjectivity and Interpretation: The flexibility of thematic analysis, while an advantage, can also be a disadvantage. The method’s openness can lead to a wide range of interpretations of the same data set, making it difficult to determine which aspects to emphasize. This potential subjectivity might raise concerns about the analysis’s reliability and consistency.
- Limited Interpretive Power: Unlike methods like narrative analysis or biographical approaches, thematic analysis may not capture the nuances of individual experiences or contradictions within a single account. The focus on patterns across interviews could result in overlooking unique individual perspectives.
- Oversimplification: Thematic analysis might oversimplify complex phenomena by focusing on common themes, potentially missing subtle but important variations within the data. If not carefully executed, the analysis may present a homogenous view of the data that doesn’t reflect the full range of perspectives.
- Lack of Established Theoretical Frameworks: Thematic analysis does not inherently rely on pre-existing theoretical frameworks. While this allows for inductive exploration, it can also limit the interpretive power of the analysis if not anchored within a relevant theoretical context. The absence of a theoretical foundation might make it challenging to draw meaningful and generalizable conclusions.
- Difficulty in Higher-Phase Analysis: While thematic analysis is relatively easy to initiate, the flexibility in its application can make it difficult to establish specific guidelines for higher-phase analysis1. Researchers may find it challenging to navigate the later stages of analysis and develop a coherent and insightful interpretation of the identified themes.
- Potential for Researcher Bias: As with any qualitative research method, thematic analysis is susceptible to researcher bias. Researchers’ preconceived notions and assumptions can influence how they code and interpret data, potentially leading to skewed results.
Reading List
- Braun, V., & Clarke, V. (2006). Using thematic analysis in psychology . Qualitative Research in Psychology, 3 (2), 77–101.
- Braun, V., & Clarke, V. (2013). Successful qualitative research: A practical guide for beginners. Sage.
- Braun, V., & Clarke, V. (2019). Reflecting on reflexive thematic analysi s. Qualitative Research in Sport, Exercise and Health, 11 (4), 589–597.
- Braun, V., & Clarke, V. (2021). One size fits all? What counts as quality practice in (reflexive) thematic analysis? Qualitative Research in Psychology, 18 (3), 328–352.
- Braun, V., & Clarke, V. (2021). To saturate or not to saturate? Questioning data saturation as a useful concept for thematic analysis and sample-size rationales . Qualitative Research in Sport, Exercise and Health, 13 (2), 201–216.
- Braun, V., & Clarke, V. (2022). Conceptual and design thinking for thematic analysis . Qualitative psychology , 9 (1), 3.
- Braun, V., & Clarke, V. (2022b). Thematic analysis: A practical guide . Sage.
- Braun, V., Clarke, V., & Hayfield, N. (2022). ‘A starting point for your journey, not a map’: Nikki Hayfield in conversation with Virginia Braun and Victoria Clarke about thematic analysis. Qualitative research in psychology , 19 (2), 424-445.
- Finlay, L., & Gough, B. (Eds.). (2003). Reflexivity: A practical guide for researchers in health and social sciences. Blackwell Science.
- Gibbs, G. R. (2013). Using software in qualitative analysis. In U. Flick (ed.) The Sage handbook of qualitative data analysis (pp. 277–294). London: Sage.
- McLeod, S. (2024, May 17). Qualitative Data Coding . Simply Psychology. https://www.simplypsychology.org/qualitative-data-coding.html
- Terry, G., & Hayfield, N. (2021). Essentials of thematic analysis . American Psychological Association.
- Trainor, L. R., & Bundon, A. (2021). Developing the craft: Reflexive accounts of doing reflexive thematic analysis . Qualitative research in sport, exercise and health , 13 (5), 705-726.
Examples of Good Practice
- Anderson, S., Clarke, V., & Thomas, Z. (2023). The problem with picking: Permittance, escape and shame in problematic skin picking . Psychology and Psychotherapy: Theory, Research and Practice , 96 (1), 83-100.
- Braun, V., Terry, G., Gavey, N., & Fenaughty, J. (2009). ‘ Risk’and sexual coercion among gay and bisexual men in Aotearoa/New Zealand–key informant accounts . Culture, Health & Sexuality , 11 (2), 111-124.
- Clarke, V., & Kitzinger, C. (2004). Lesbian and gay parents on talk shows: resistance or collusion in heterosexism? . Qualitative Research in Psychology , 1 (3), 195-217.
- Hayfield, N., Jones, B., Carter, J., & Jowett, A. (2024). Exploring civil partnership from the perspective of those in mixed-sex relationships: Embracing a clean slate of equality . Journal of Family Issues , 45 (8), 1925-1948.
- Hayfield, N., Moore, H., & Terry, G. (2024). “Friends? Supported. Partner? Not so much…”: Women’s experiences of friendships, family, and relationships during perimenopause and menopause . Feminism & Psychology , 09593535241242563.
- Lovell, D., Hayfield, N., & Thomas, Z. (2023). “No one has ever asked me and I’m grateful that you have” men’s experiences of their partner’s female sexual pain . Sexual and Relationship Therapy , 1-24.
- Wheeler, L., Fragkiadaki, E., Clarke, V., & DiCaccavo, A. (2022). ‘Sunshine’,‘angels’ and ‘rainbows’: language developed by mothers bereaved by perinatal loss. British Journal of Midwifery , 30 (7), 368-374.
- Answers to frequently asked questions about thematic analysis
- Thematic analysis – data for coding exercise
- University of Auckland – Thematic Analysis Resources

- How it works
"Christmas Offer"
Terms & conditions.
As the Christmas season is upon us, we find ourselves reflecting on the past year and those who we have helped to shape their future. It’s been quite a year for us all! The end of the year brings no greater joy than the opportunity to express to you Christmas greetings and good wishes.
At this special time of year, Research Prospect brings joyful discount of 10% on all its services. May your Christmas and New Year be filled with joy.
We are looking back with appreciation for your loyalty and looking forward to moving into the New Year together.
"Claim this offer"
In unfamiliar and hard times, we have stuck by you. This Christmas, Research Prospect brings you all the joy with exciting discount of 10% on all its services.
Offer valid till 5-1-2024
We love being your partner in success. We know you have been working hard lately, take a break this holiday season to spend time with your loved ones while we make sure you succeed in your academics
Discount code: RP0996Y
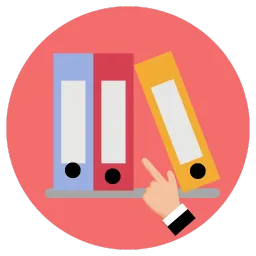
Thematic Analysis – A Guide with Examples
Published by Alvin Nicolas at August 16th, 2021 , Revised On August 29, 2023
Thematic analysis is one of the most important types of analysis used for qualitative data . When researchers have to analyse audio or video transcripts, they give preference to thematic analysis. A researcher needs to look keenly at the content to identify the context and the message conveyed by the speaker.
Moreover, with the help of this analysis, data can be simplified.
Importance of Thematic Analysis
Thematic analysis has so many unique and dynamic features, some of which are given below:
Thematic analysis is used because:
- It is flexible.
- It is best for complex data sets.
- It is applied to qualitative data sets.
- It takes less complexity compared to other theories of analysis.
Intellectuals and researchers give preference to thematic analysis due to its effectiveness in the research.
How to Conduct a Thematic Analysis?
While doing any research , if your data and procedure are clear, it will be easier for your reader to understand how you concluded the results . This will add much clarity to your research.
Understand the Data
This is the first step of your thematic analysis. At this stage, you have to understand the data set. You need to read the entire data instead of reading the small portion. If you do not have the data in the textual form, you have to transcribe it.
Example: If you are visiting an adult dating website, you have to make a data corpus. You should read and re-read the data and consider several profiles. It will give you an idea of how adults represent themselves on dating sites. You may get the following results:
I am a tall, single(widowed), easy-going, honest, good listener with a good sense of humor. Being a handyperson, I keep busy working around the house, and I also like to follow my favourite hockey team on TV or spoil my two granddaughters when I get the chance!! Enjoy most music except Rap! I keep fit by jogging, walking, and bicycling (at least three times a week). I have travelled to many places and RVD the South-West U.S., but I would now like to find that special travel partner to do more travel to warm and interesting countries. I now feel it’s time to meet a nice, kind, honest woman who has some of the same interests as I do; to share the happy times, quiet times, and adventures together
I enjoy photography, lapidary & seeking collectibles in the form of classic movies & 33 1/3, 45 & 78 RPM recordings from the 1920s, ’30s & ’40s. I am retired & looking forward to travelling to Canada, the USA, the UK & Europe, China. I am unique since I do not judge a book by its cover. I accept people for who they are. I will not demand or request perfection from anyone until I am perfect, so I guess that means everyone is safe. My musical tastes range from Classical, big band era, early jazz, classic ’50s & 60’s rock & roll & country since its inception.
Development of Initial Coding:
At this stage, you have to do coding. It’s the essential step of your research . Here you have two options for coding. Either you can do the coding manually or take the help of any tool. A software named the NOVIC is considered the best tool for doing automatic coding.
For manual coding, you can follow the steps given below:
- Please write down the data in a proper format so that it can be easier to proceed.
- Use a highlighter to highlight all the essential points from data.
- Make as many points as possible.
- Take notes very carefully at this stage.
- Apply themes as much possible.
- Now check out the themes of the same pattern or concept.
- Turn all the same themes into the single one.
Example: For better understanding, the previously explained example of Step 1 is continued here. You can observe the coded profiles below:
Make Themes
At this stage, you have to make the themes. These themes should be categorised based on the codes. All the codes which have previously been generated should be turned into themes. Moreover, with the help of the codes, some themes and sub-themes can also be created. This process is usually done with the help of visuals so that a reader can take an in-depth look at first glance itself.
Extracted Data Review
Now you have to take an in-depth look at all the awarded themes again. You have to check whether all the given themes are organised properly or not. It would help if you were careful and focused because you have to note down the symmetry here. If you find that all the themes are not coherent, you can revise them. You can also reshape the data so that there will be symmetry between the themes and dataset here.
For better understanding, a mind-mapping example is given here:
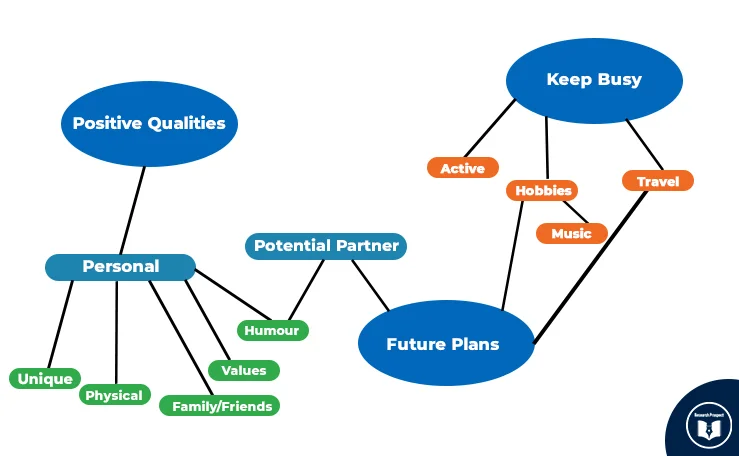
Reviewing all the Themes Again
You need to review the themes after coding them. At this stage, you are allowed to play with your themes in a more detailed manner. You have to convert the bigger themes into smaller themes here. If you want to combine some similar themes into a single theme, then you can do it. This step involves two steps for better fragmentation.
You need to observe the coded data separately so that you can have a precise view. If you find that the themes which are given are following the dataset, it’s okay. Otherwise, you may have to rearrange the data again to coherence in the coded data.
Corpus Data
Here you have to take into consideration all the corpus data again. It would help if you found how themes are arranged here. It would help if you used the visuals to check out the relationship between them. Suppose all the things are not done accordingly, so you should check out the previous steps for a refined process. Otherwise, you can move to the next step. However, make sure that all the themes are satisfactory and you are not confused.
When all the two steps are completed, you need to make a more précised mind map. An example following the previous cases has been given below:
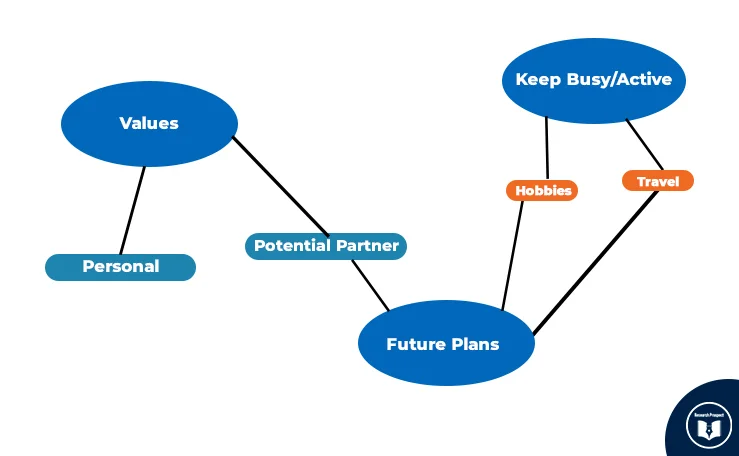
Define all the Themes here
Now you have to define all the themes which you have given to your data set. You can recheck them carefully if you feel that some of them can fit into one concept, you can keep them, and eliminate the other irrelevant themes. Because it should be precise and clear, there should not be any ambiguity. Now you have to think about the main idea and check out that all the given themes are parallel to your main idea or not. This can change the concept for you.
The given names should be so that it can give any reader a clear idea about your findings. However, it should not oppose your thematic analysis; rather, everything should be organised accurately.
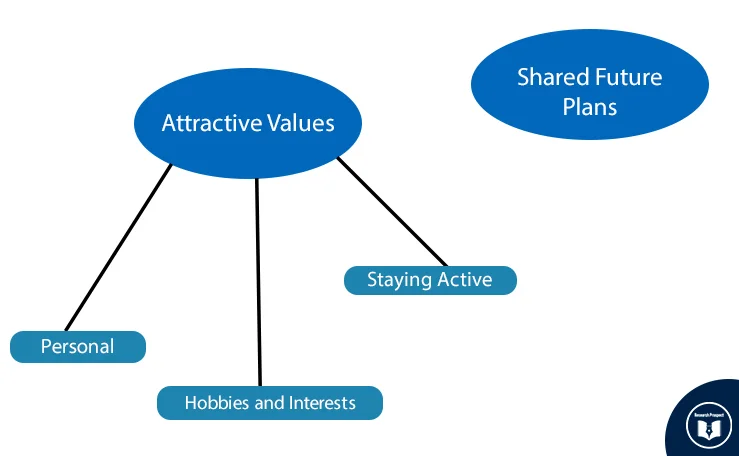
Does your Research Methodology Have the Following?
- Great Research/Sources
- Perfect Language
- Accurate Sources
If not, we can help. Our panel of experts makes sure to keep the 3 pillars of Research Methodology strong.
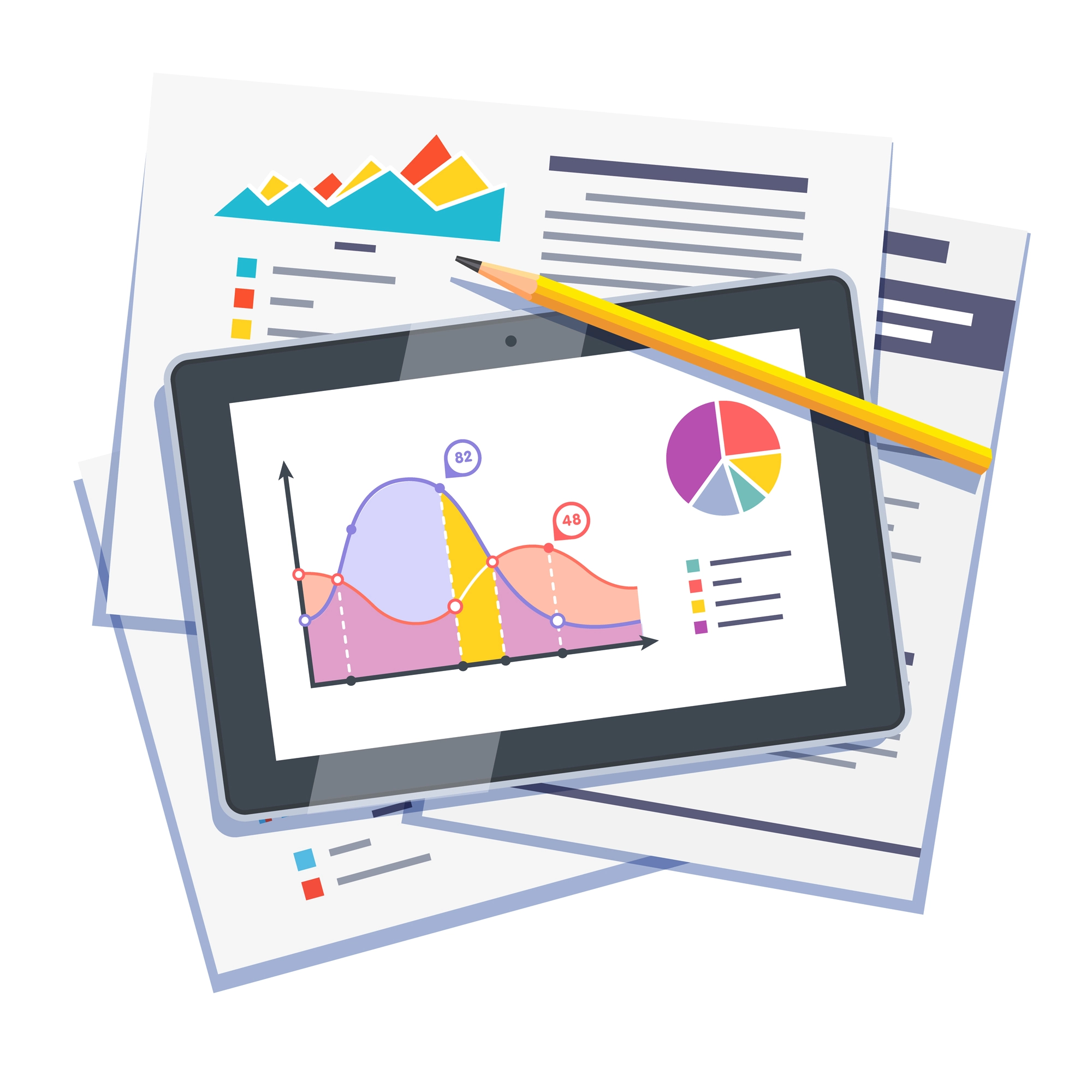
Also, read about discourse analysis , content analysis and survey conducting . we have provided comprehensive guides.
Make a Report
You need to make the final report of all the findings you have done at this stage. You should include the dataset, findings, and every aspect of your analysis in it.
While making the final report , do not forget to consider your audience. For instance, you are writing for the Newsletter, Journal, Public awareness, etc., your report should be according to your audience. It should be concise and have some logic; it should not be repetitive. You can use the references of other relevant sources as evidence to support your discussion.
Frequently Asked Questions
What is meant by thematic analysis.
Thematic Analysis is a qualitative research method that involves identifying, analyzing, and interpreting recurring themes or patterns in data. It aims to uncover underlying meanings, ideas, and concepts within the dataset, providing insights into participants’ perspectives and experiences.
You May Also Like
Struggling to figure out “whether I should choose primary research or secondary research in my dissertation?” Here are some tips to help you decide.
What are the different research strategies you can use in your dissertation? Here are some guidelines to help you choose a research strategy that would make your research more credible.
This article presents the key advantages and disadvantages of secondary research so you can select the most appropriate research approach for your study.
As Featured On
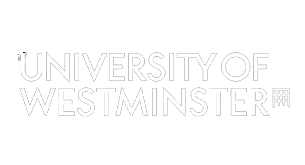
USEFUL LINKS
LEARNING RESOURCES
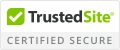
COMPANY DETAILS
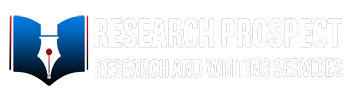
Splash Sol LLC

- How It Works

The Guide to Thematic Analysis
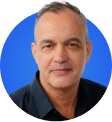
- Introduction
Thematic analysis explained
What's unique about thematic analysis, different approaches to thematic analysis.
- The mini-guide to thematic analysis
- Advantages of Thematic Analysis
- Disadvantages of Thematic Analysis
- Thematic Analysis Examples
- How to Do Thematic Analysis
- Thematic Coding
- Collaborative Thematic Analysis
- Thematic Analysis Software
- Thematic Analysis in Mixed Methods Approach
- Abductive Thematic Analysis
- Deductive Thematic Analysis
- Inductive Thematic Analysis
- Reflexive Thematic Analysis
- Thematic Analysis in Observations
- Thematic Analysis in Surveys
- Thematic Analysis for Interviews
- Thematic Analysis for Focus Groups
- Thematic Analysis for Case Studies
- Thematic Analysis of Secondary Data
- Thematic Analysis Literature Review
- Thematic Analysis vs. Phenomenology
- Thematic vs. Content Analysis
- Thematic Analysis vs. Grounded Theory
- Thematic Analysis vs. Narrative Analysis
- Thematic Analysis vs. Discourse Analysis
- Thematic Analysis vs. Framework Analysis
- Thematic Analysis in Social Work
- Thematic Analysis in Psychology
- Thematic Analysis in Educational Research
- Thematic Analysis in UX Research
- How to Present Thematic Analysis Results
- Increasing Rigor in Thematic Analysis
- Peer Review in Thematic Analysis
What is Thematic Analysis?
Thematic analysis is a central method in qualitative research used to identify patterns within data. Under a thematic analysis paradigm, researchers analyze qualitative data to organize and describe their dataset in detail through themes and motifs that emerge from the data itself. This approach is flexible and can be applied across a wide range of social science fields, accommodating various datasets and research questions . The technique does not subscribe to a rigid framework, allowing for adaptation to the specific needs of the study. Thematic analysis is valuable for its ability to unearth nuanced insights into complex data sets, providing a structured yet adaptable tool for qualitative analysis .
This guide will outline the advantages and disadvantages of thematic analysis, discuss its different types and their processes, and showcase its application in diverse social science disciplines.
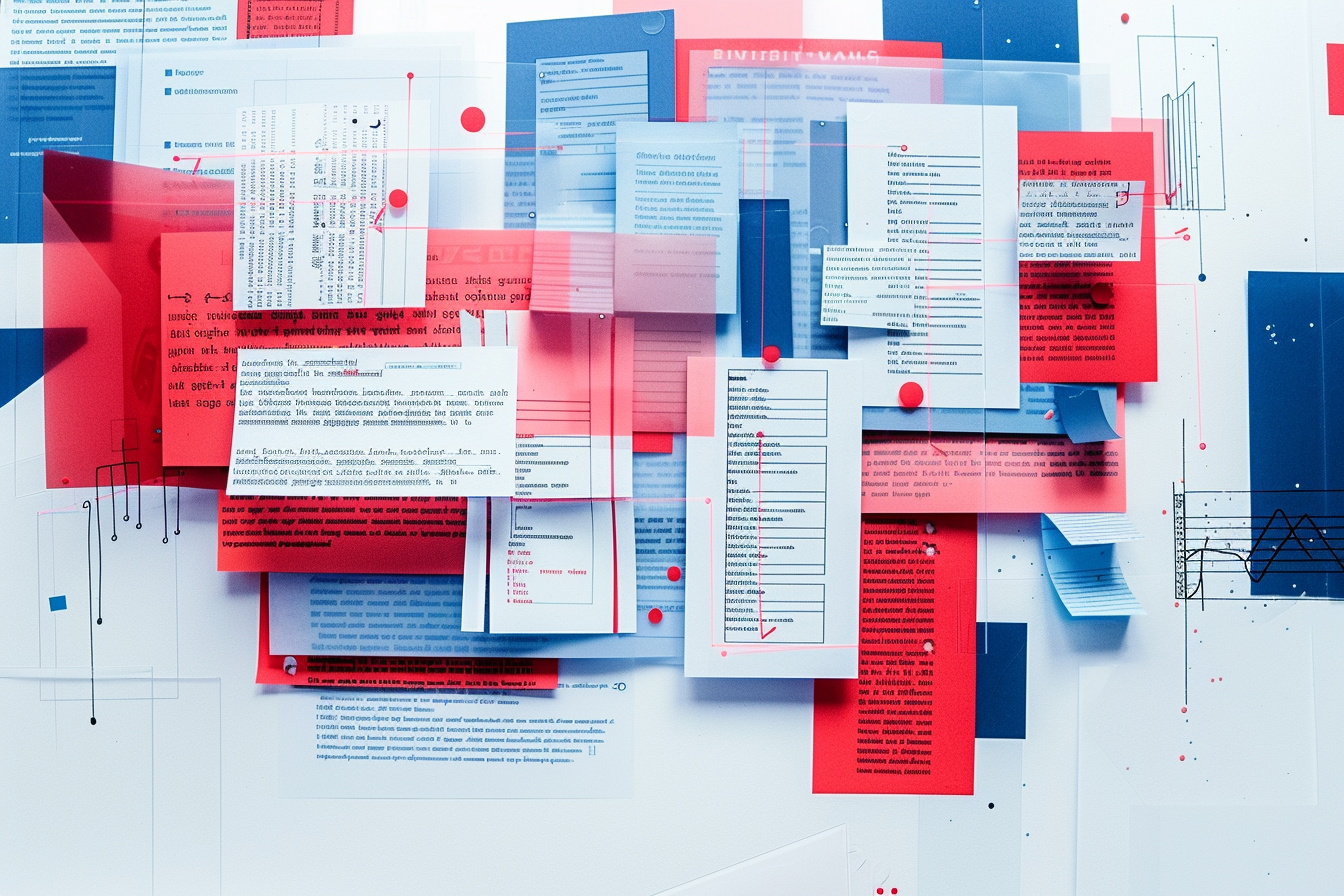
Thematic analysis approaches are among the most flexible methods accessible to both expert and novice researchers. Thematic analysis emphasizes the data itself, enabling researchers to derive significant insights directly from their collected information.
But what is it and what role does it play in analyzing qualitative data ? This section will cover the purpose of qualitative research , define qualitative data analysis, outline the thematic analysis process, and explain the necessity of thematic analysis for qualitative researchers. Through this, the significance and functionality of thematic analysis in the context of research will be clarified.
What is the purpose of qualitative research?
Qualitative research aims to understand human behavior, experiences, and the reasons that govern such behavior and experiences. Unlike quantitative research , which seeks to quantify data and generalize results from sample populations to larger populations, qualitative research focuses on understanding the depth and complexity of social phenomena, prioritizing contextualisation over generalization. This type of research is interested in the 'how' and 'why' questions, seeking to provide insights into problems, develop ideas or hypotheses for potential quantitative research, and uncover trends in thought and opinions.
Qualitative data collection methods include interviews , focus groups , and observational research , among others. Each method is chosen based on its ability to provide the most meaningful and relevant data for the research question at hand. The primary goal is to gain a detailed and nuanced understanding of people's attitudes, behaviors, and interactions in their natural settings.
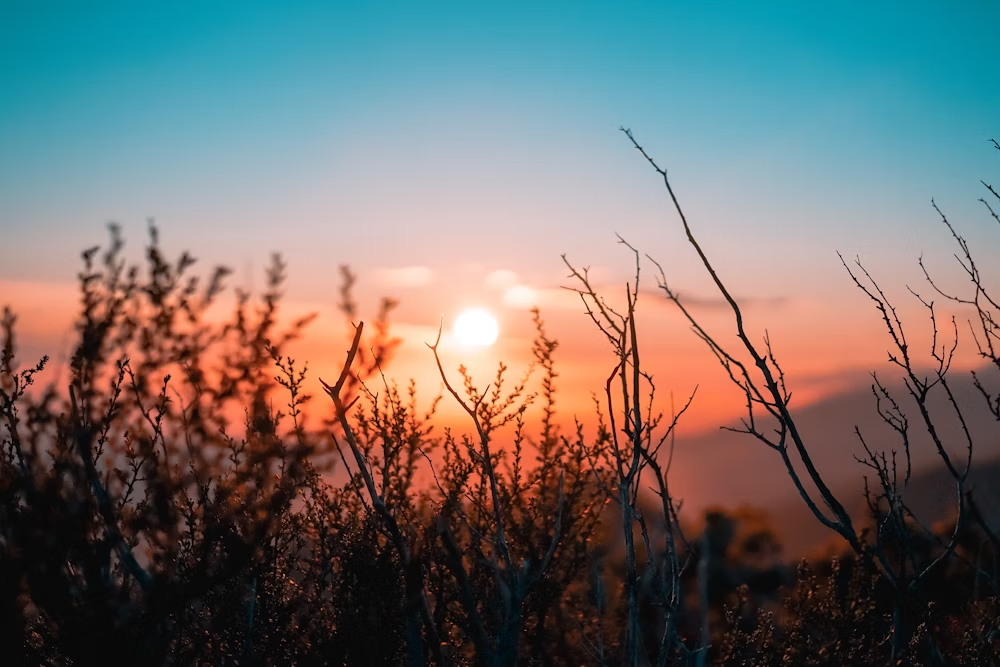
What is qualitative data analysis?
Qualitative data analysis is the process of systematically examining non-numerical data (e.g., text, video, or audio) to understand meanings, patterns, and relationships. While quantitative data is more accessible through statistical analysis, qualitative data analysis transforms raw data into findings through a meticulous process of coding and identifying themes or patterns.
The analysis begins with data collection, followed by reading and re-reading the data to gain a deep familiarity with its content. Researchers then proceed to data coding by applying tags or labels that categorize segments of the data into meaningful groups for further analysis. These codes are refined and grouped into themes that capture the essence of the entire data set. A theme is a pattern within the data that represents a significant aspect of the research question or provides insight into the dataset.
Qualitative data analysis is iterative, requiring researchers to move back and forth between the dataset and the emerging analysis to ensure that the themes accurately represent the data. This approach allows for the identification of subtleties and complexities within the data that might not be apparent on the surface. The process is critical for developing a comprehensive understanding of the context, motivations, and experiences of research subjects.
Qualitative data analysis refers to a whole host of analytical approaches such as narrative analysis , discourse analysis , and qualitative content analysis . Each provides a systematic and rigorous approach for uncovering and interpreting the richness and diversity of data, and what is important is that the analytical approach fits with the research question . Through the analysis process, researchers can construct a coherent narrative that not only addresses their research questions but also adds depth and dimension to their findings.
What is the thematic analysis process?
The thematic analysis process involves several stages to ensure the thorough examination and interpretation of qualitative data. This process typically begins with the collection of data, which can be in the form of interviews, focus groups, observations, or textual and visual materials. As data collection begins, the thematic analysis process follows these steps:
- Familiarization with the data : Researchers immerse themselves in the data, reading and re-reading the material, and possibly taking initial notes. This stage is essential for understanding the breadth and depth of the content.
- Generating initial codes : Through a careful examination of the data, researchers identify specific elements that appear interesting and code them accordingly. Coding involves tagging segments of the data with labels that summarize or account for each piece of information. This process helps in organizing the data into manageable chunks for further analysis.
- Searching for themes : Codes are then reviewed and grouped together into potential themes. A theme captures something important about the data in relation to the research question and represents some level of patterned response or meaning within the data set.
- Reviewing themes : This step involves refining the themes to ensure they work in relation to the coded extracts and the entire data set. It may involve splitting, combining, or discarding themes.
- Defining and naming themes : Once a set of coherent themes has been established, each theme is clearly defined and named. This involves identifying the essence of what each theme is about and determining what aspect of the data each theme captures.
- Producing the report : The final step involves weaving together the analytical narrative and data extracts, and contextualizing the analysis in relation to existing literature and the research question. A typical thematic analysis report should present a coherent and logical story that convinces the reader that the chosen themes reflect the data and address the research question.
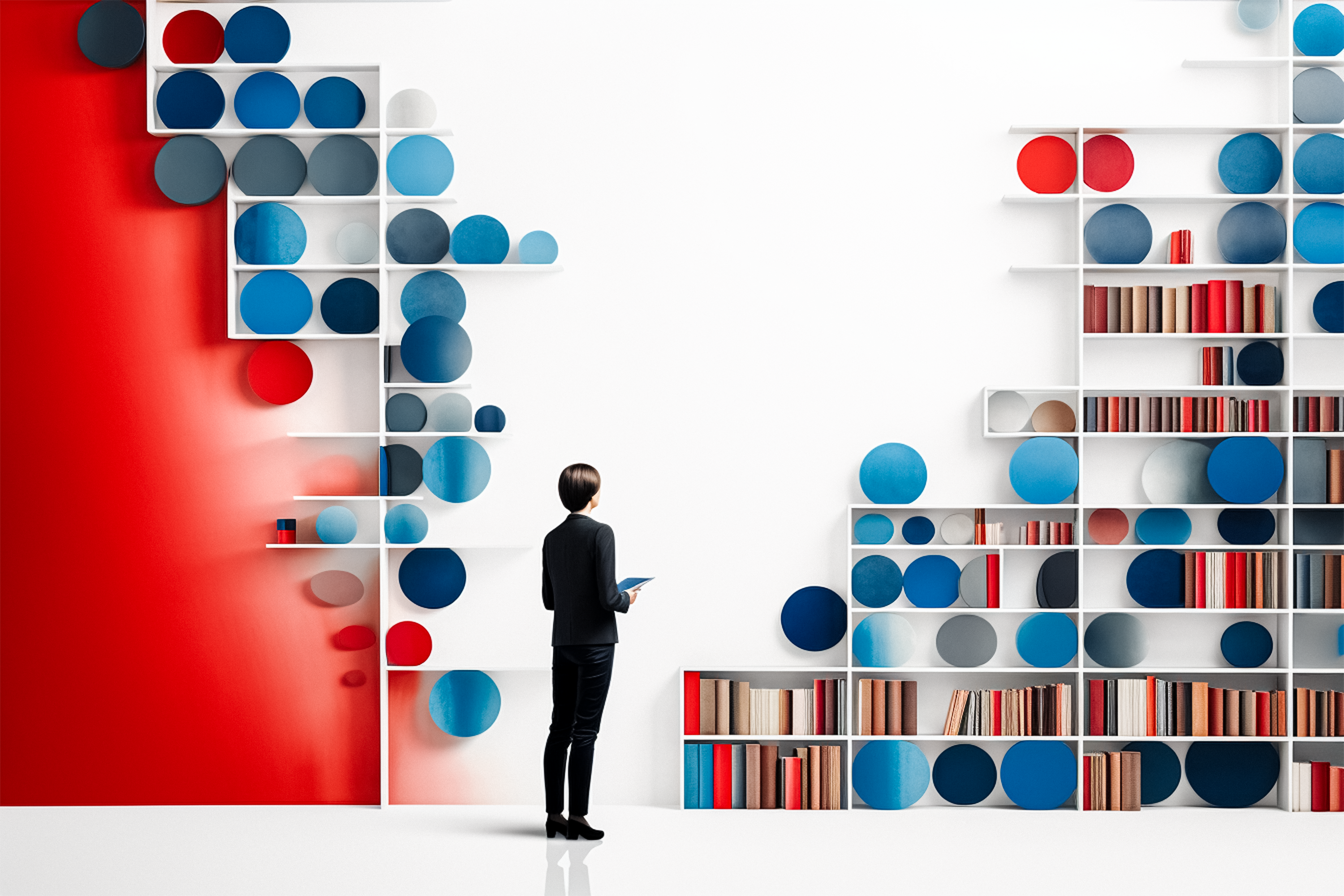
Why do qualitative researchers need thematic analysis?
Thematic analysis offers qualitative researchers a flexible yet systematic approach for examining data. This method is not tied to any specific theoretical framework , allowing researchers to apply it across a wide range of epistemologies and research questions. Here are several reasons why thematic analysis is indispensable for qualitative researchers:
- Versatility : It can be used with various data types, including interview transcripts , survey responses , and social media content, making it applicable to many research fields.
- Depth of insight : Thematic analysis allows researchers to examine the complexities and nuances of their data, providing a rich, detailed, and complex account of their findings.
- Accessibility : The process is accessible to researchers at different levels of experience, from beginners to experts, due to its clear and systematic nature.
- Transparency : By providing a structured approach to data analysis, thematic analysis enhances the transparency of qualitative research. Researchers can clearly demonstrate how their findings are derived from the data, which contributes to the credibility and trustworthiness of the research.
- Comprehensiveness : It enables researchers to examine large volumes of data in a manageable way, ensuring that potential insights are not overlooked.
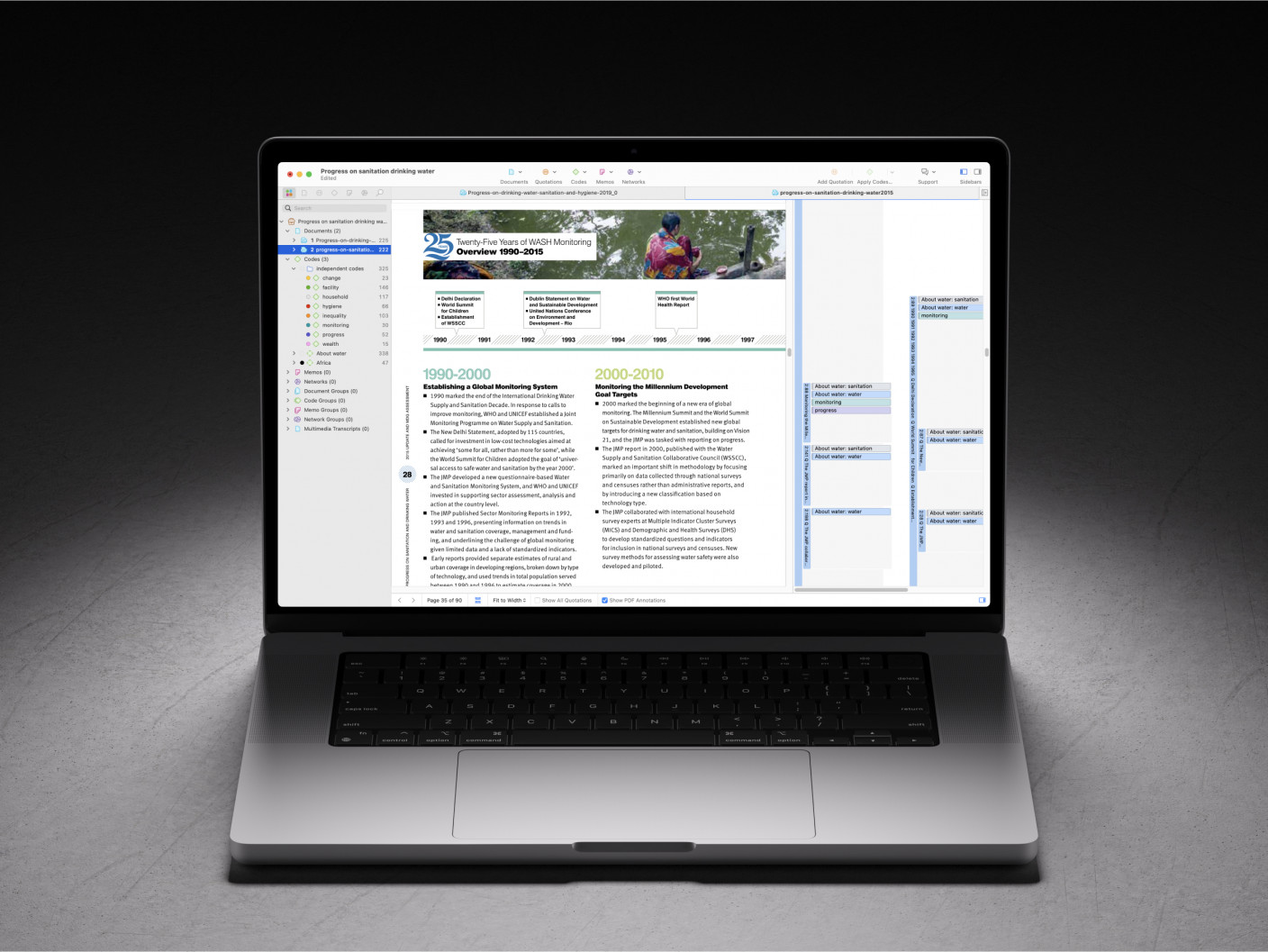
ATLAS.ti makes the most of your qualitative data
Turn to our powerful data analysis tools to deliver critical insights from your research. Download a free trial today.
Advantages with conducting thematic analysis center on its ease of use. In particular, thematic analysis is flexible, accessible, and can be applied to many different fields. When compared to other qualitative methods such as discourse analysis, narrative analysis, and content analysis, thematic analysis offers distinct advantages and features that cater to a wide range of research needs and questions.
Discourse analysis
Discourse analysis focuses on the way language is used in texts and conversations to construct meanings and social realities. It pays close attention to the context in which language is used and how power relations and identities are constructed through discourse. In contrast, thematic analysis takes a broader view of the data beyond (but including) language and discourse. This makes thematic analysis more versatile and applicable to a broader variety of data types, not just textual or conversational data.
Narrative analysis
Narrative analysis looks into the storytelling aspects of data, exploring how individuals construct and convey their experiences and realities through narratives. This approach is particularly focused on the structure and function of stories within the data, examining how these narratives help individuals make sense of their world. Thematic analysis, by contrast, is less concerned with the form or structure of narratives and more focused on identifying and analyzing themes that cut across the data, regardless of how they are narrated.
Content analysis
Content analysis is a method that quantifies content in terms of predetermined categories and often involves counting the frequency of words, themes, or concepts within the data. While content analysis provides a systematic way to analyze textual data, it tends to focus more on surface-level aspects of the data and less interpretative analyses compared to thematic analysis. Thematic analysis goes beyond mere counting or categorization to interpret the underlying ideas, assumptions, and conceptualizations within the data.
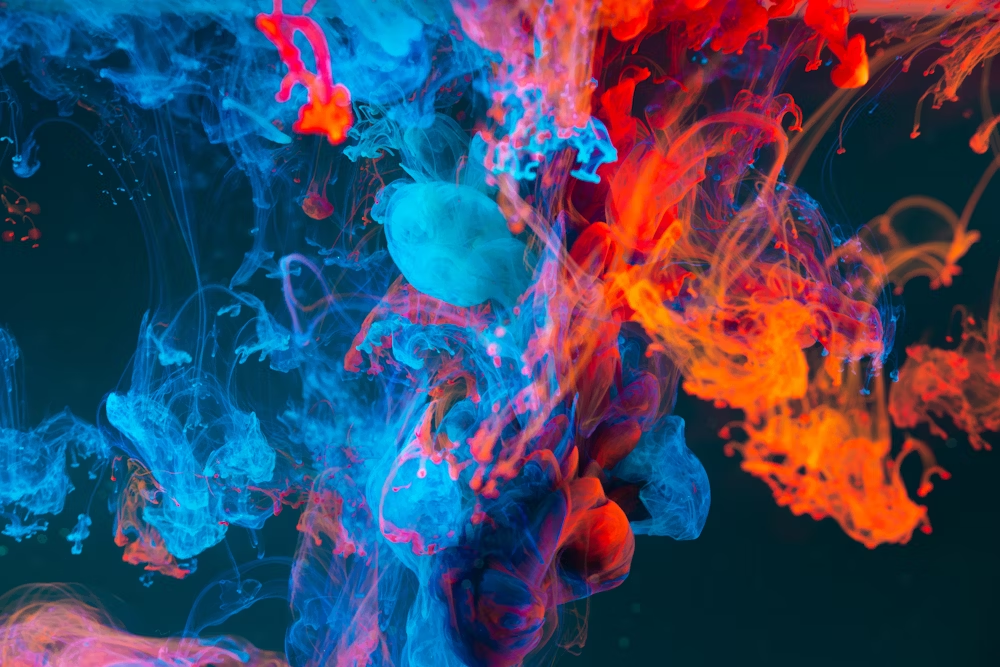
Thematic analysis is a flexible method for qualitative research , accommodating various approaches based on the researcher's objectives, theoretical framework , and the nature of the data . Three notable approaches are inductive thematic analysis, deductive thematic analysis, and reflexive thematic analysis. Each approach has distinct characteristics and applications, tailored to specific research needs.
Inductive thematic analysis
Inductive thematic analysis is driven by the data itself, rather than being guided by pre-existing theories or researcher expectations. This bottom-up approach allows themes to emerge directly from the data, with coding and theme development rooted in the content of the dataset. Inductive analysis is particularly useful when exploring new or under-researched areas where the researcher aims to gain fresh insights without the constraints of existing theoretical frameworks.
Deductive thematic analysis
Deductive thematic analysis, in contrast, is a top-down approach where the researcher starts with pre-defined codes or theoretical concepts that guide the analysis. This method is applied when the research is framed by specific theories or when the study aims to examine particular aspects of the data. Deductive analysis ensures that the investigation remains closely aligned with the research questions or hypotheses that are based on the literature or theoretical considerations. This approach can provide a focused examination of the data, allowing for a targeted exploration of predefined themes.
Reflexive thematic analysis
Reflexive thematic analysis emphasizes the active role of the researcher in the analysis process. It involves continuous reflection on the way researchers' biases, assumptions, and backgrounds influence the interpretation of the data. Reflexive thematic analysis is not strictly inductive or deductive but is characterized by a constant dialogue between the researcher, the data, and the emerging analysis. This approach acknowledges the subjective nature of the analysis and seeks to make the research process as transparent as possible, allowing for a nuanced and in-depth understanding of the data.
The guide to thematic analysis
Thematic analysis is a flexible method for gathering key insights from qualitative data , and it merits a comprehensive discussion to cover all the important points that facilitate a rigorous analysis and transparent research inquiry . That's why we've written this guide to provide you with a foundational understanding of thematic analysis that you can apply to your qualitative research.
We've divided this guide into several sections, which we've outlined below. If you are new to thematic analysis or to qualitative research , we suggest reading these articles in order so you can get a sense of not only the process of conducting thematic analysis, but the reasoning behind this methodological approach. However, if you simply need a refresher on certain aspects of thematic analysis, feel free to navigate to the article that is most appropriate for you.
Introduction to thematic analysis
In this section, we'll explore the advantages and disadvantages of thematic analysis.
Thematic analysis basics
This section provides some basic information about thematic analysis through examples and some important aspects to keep in mind when applying thematic analysis to your research.
Types of thematic analysis
There are many kinds of thematic analysis to consider, so we'll look at each of the major types of thematic analysis in this section.
Thematic analysis with qualitative data collection
Qualitative data collection takes on various forms, so it will be important to understand how to apply thematic analysis to each of the major types of qualitative data.
Thematic analysis with other forms of qualitative analysis
What is the best analytical approach for your research? In this section, we'll compare thematic analysis to other analytic methods in qualitative research.
Research fields using thematic analysis
How is thematic analysis applied in various social science fields? We'll explore its use in four different research areas in this section.
Publishing thematic analysis research
After you analyze qualitative data, your job is to persuade your audience of its impact. We'll discuss this in the following articles.
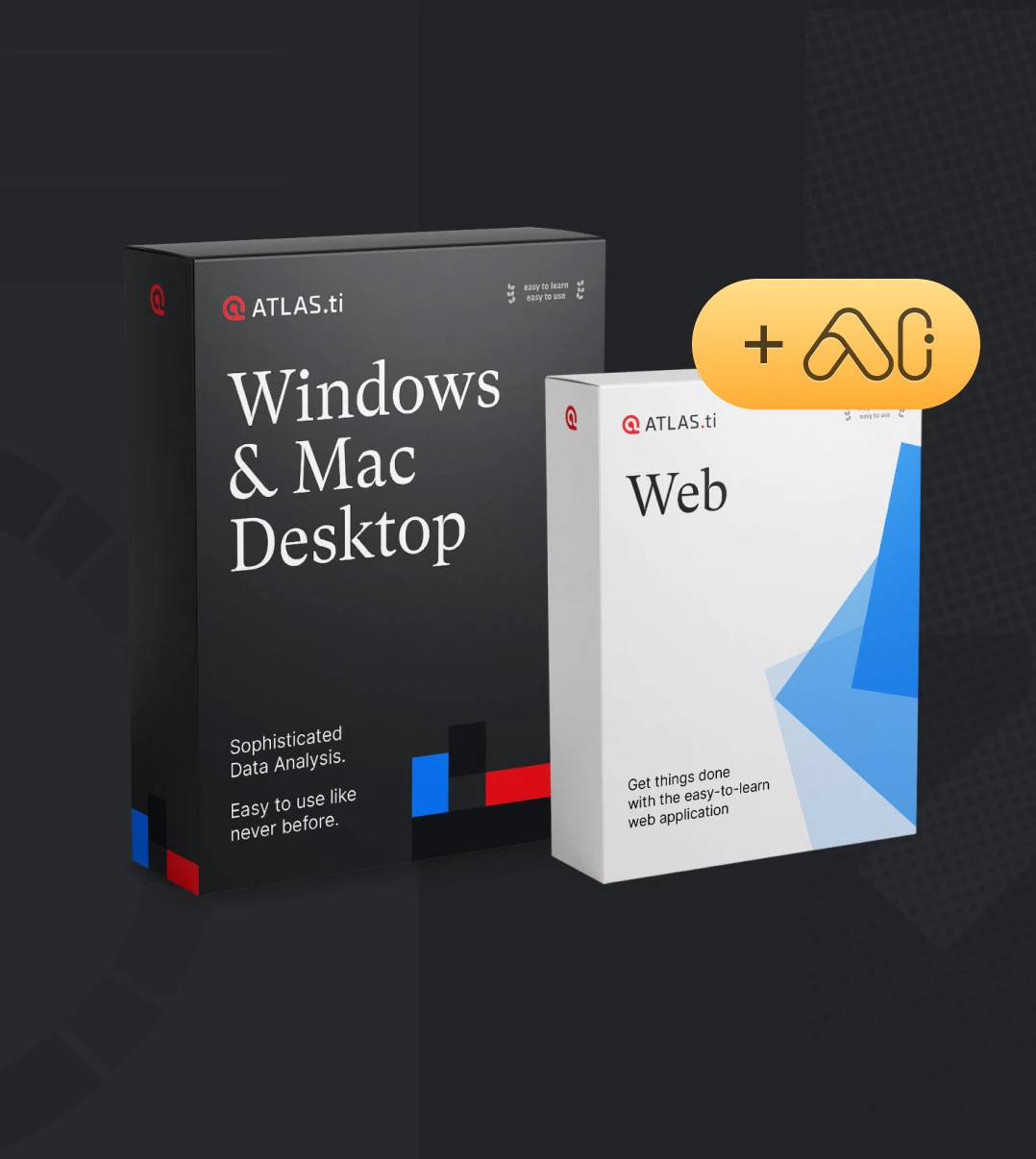
From initial analysis to final report, turn to ATLAS.ti
Our tools are the solution for your qualitative analysis needs. See why with a free trial.

IMAGES
VIDEO