- The Future of Mass Spectrometry
- Separation Science - The State of The Art in Life Science Analysis
- PFAS Focus 2024: Water Analysis
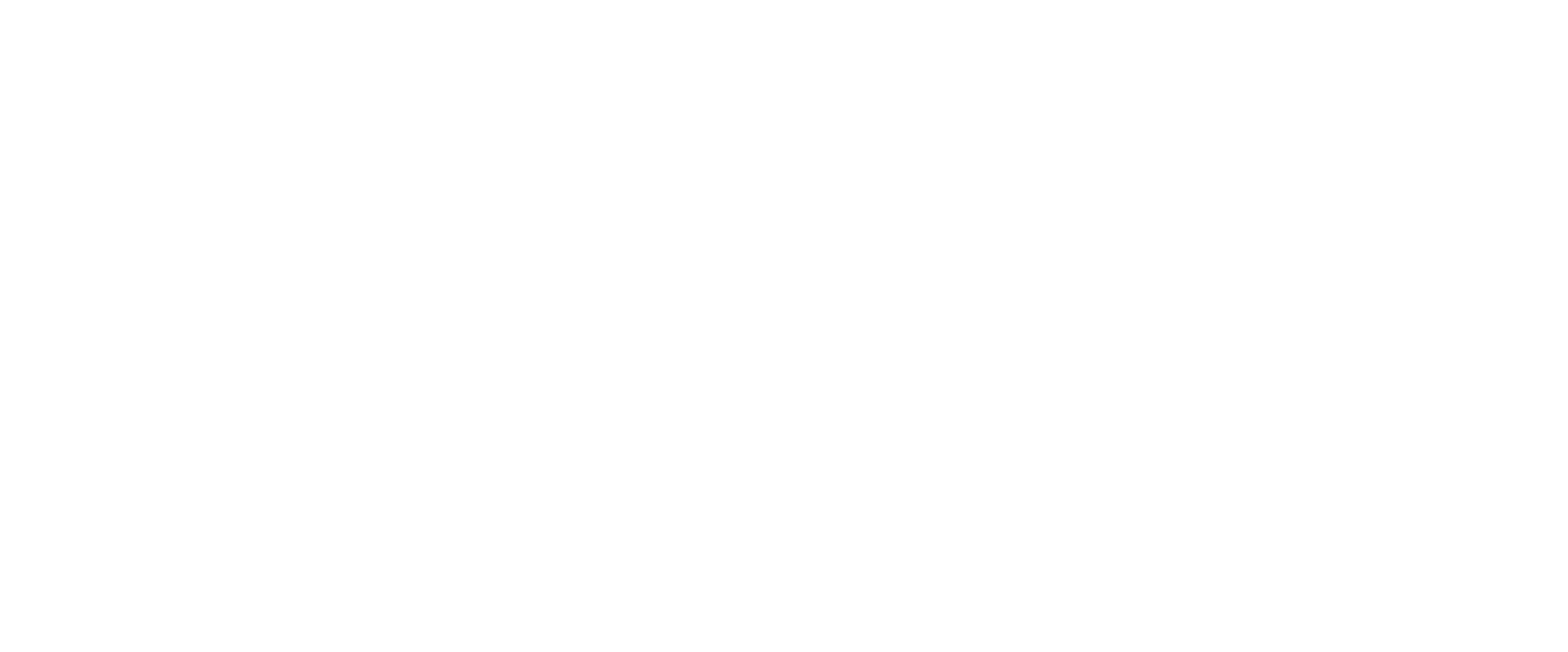
- Publications
- Conferences

Tips for Troubleshooting Liquid–Liquid Extractions
LCGC North America
A common challenge in LLE is the formation of emulsions. Several approaches can address the problem. Or, one can try an alternative technique.
Liquid–liquid extraction (LLE) is the most widely used extraction technique for liquid samples. The information provided in this article focuses on LLE using a separatory funnel. Some of the potential issues that may require troubleshooting when using LLE include (1):
- Emulsion formation
- Analytes strongly adsorbing to particulates
- Analytes binding to high-molecular-weight compounds (protein–drug interactions)
- Mutual solubility of the two phases
- Potential for solvent mediated decomposition
- Method robustness and transfer issues because of manual processing
The formation of emulsions is at the top of the list because it is a very common problem. Let's focus on how to troubleshoot and avoid this issue, as well as offer an alternative extraction technique that precludes emulsion formation.
Emulsions commonly occur when a sample contains a high amount of surfactant-like compounds (that is, phospholipids, free fatty acids, triglycerides, proteins, and so on). These surfactant-like molecules are large and will have mutual solubility in the aqueous and organic solvents which results in the formation of an emulsion in a mid-zone between the two phases. This intermediate solubility in each of the two phases makes it difficult to quantitatively collect one phase or another. Furthermore, the emulsion can also trap some of the analyte of interest, leading to quantitative problems. Emulsions often occur with samples where the animal (or human) diet is high in fats. Thus, emulsions sometimes appear when passing from preclinical trials with animals on low-fat controlled diets to clinical trials with humans who may be on high-fat diets. This characteristic problem makes LLE a less dependable procedure if it is expected that the same extraction protocol will be used for both preclinical and clinical samples. If this problem is anticipated, it is worth trying high-fat samples during method development in addition to the standard test matrices.
There are a few tricks of the trade to either stop emulsions from forming in the first place or to disrupt them if they do form. As a general rule, it is easier to prevent emulsion formation than to break it after one has formed. The simplest way to prevent the formation of an emulsion is to gently swirl instead of shake the separatory funnel. By swirling the separatory funnel the agitation that can cause the emulsion to form is reduced, but the surface area of contact between the two phases is maintained to allow for extraction to occur.
Emulsions can be disrupted by the addition of brine or salt water, which increases the ionic strength of the aqueous layer and facilitates separation of the two phases by forcing the surfactant-like molecule to separate into one phase or the other-this technique is known as salting out .
The individual layers or emulsion can often be separated via filtration through a glass wool plug (to remove the emulsion) or a phase separation filter paper (to isolate a specific layer). Phase separation filter papers are highly silanized and, depending on the type of paper, will allow either the aqueous or organic phase to pass through and be isolated. Centrifugation of the separation can also be used to isolate the emulsion material in the residue of the centrifugation.
Addition of a small amount of a different organic solvent will adjust the solvent properties of the separation and can result in the surfactant-like molecule being solubilized in either the organic or aqueous layer to a greater extent, which breaks the emulsion.
Supported liquid extraction (SLE) is a technique that can be used for samples that are prone to emulsion formation (2). Analytes are separated based on differential solubility. The aqueous sample may be pretreated-for example, the pH can be adjusted so that the analytes are in a suitable form to be extracted into an organic solvent. Following this adjustment, the sample is applied to a solid support (often diatomaceous earth), which creates an interface for extraction. A small volume of water-immiscible organic solvent is subsequently passed over the matrix holding the aqueous layer and the analytes partition into the organic phase. The extraction solvent is allowed to percolate by gravity; sometimes a gentle vacuum or pressure is applied. Organic solvents that are commonly used include ethyl acetate, methyl tert -butyl ether (MTBE), dichloromethane, hexane, and mixtures thereof.
(1) http://www.chromacademy.com/chromatography-Troubleshooting-Liquid-Liquid-Extraction.html .
(2) http://www.chromacademy.com/chromatography-Supported-Liquid-Extraction-SLE.html .
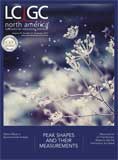
LLC-HRMS Technology Used to Track Chemical Migration in Reusable Plastic Bottles
Aiming to develop a comprehensive strategy for evaluating the chemical migration from various reusable plastic bottles, researchers from the University of Copenhagen utilized vacuum evaporation concentration (VEC) enrichment combined with liquid chromatography (LC) high resolution mass spectrometry (HRMS) analysis to investigate a wide range of materials from different manufacturers to identify those with higher and lower chemical migration rates.
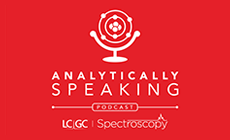
Ep. 30: The Potential of Generative Artificial Intelligence as a Research Assistant for Measurement Science
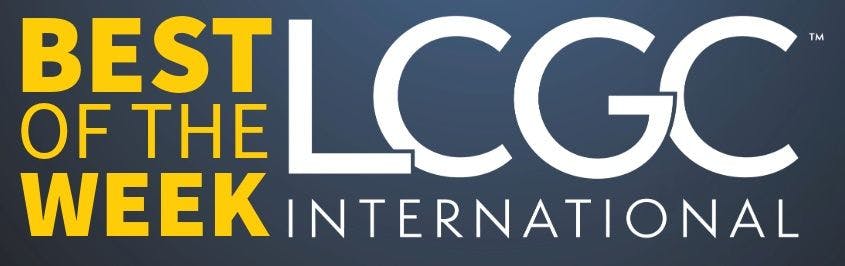
Best of the Week: Emerging Chromatography Leader, Bladder Cancer Research, and HPLC 2025
Here is some of the most popular content posted on LCGC International this week.

AI and GenAI Applications to Help Optimize Purification and Yield of Antibodies From Plasma
Deriving antibodies from plasma products involves several steps, typically starting from the collection of plasma and ending with the purification of the desired antibodies. These are: plasma collection; plasma pooling; fractionation; antibody purification; concentration and formulation; quality control; and packaging and storage. This process results in a purified antibody product that can be used for therapeutic purposes, diagnostic tests, or research. Each step is critical to ensure the safety, efficacy, and quality of the final product. Applications of AI/GenAI in many of these steps can significantly help in the optimization of purification and yield of the desired antibodies. Some specific use-cases are: selecting and optimizing plasma units for optimized plasma pooling; GenAI solution for enterprise search on internal knowledge portal; analysing and optimizing production batch profitability, inventory, yields; monitoring production batch key performance indicators for outlier identification; monitoring production equipment to predict maintenance events; and reducing quality control laboratory testing turnaround time.

In Bruges: HPLC 2025
The 54th International Symposium on High Performance Liquid Phase Separations and Related Techniques (HPLC 2025) will be held from Sunday through Thursday, June 15-19, 2025, in Bruges, Belgium.

RP-HPLC Analysis of Polyphenols and Antioxidants in Dark Chocolate
A recent study set out to assess the significance of geographical and varietal factors in the content of alkaloids, phenolic compounds, and the antioxidant capacity of chocolate samples. Filtered extracts were analyzed by reversed-phase high-performance liquid chromatography (RP-HPLC) with ultraviolet (UV) and spectrophotometric methods to determine individual phenolics and overall indexes of antioxidant and flavonoid content.
2 Commerce Drive Cranbury, NJ 08512
609-716-7777


- Science Notes Posts
- Contact Science Notes
- Todd Helmenstine Biography
- Anne Helmenstine Biography
- Free Printable Periodic Tables (PDF and PNG)
- Periodic Table Wallpapers
- Interactive Periodic Table
- Periodic Table Posters
- Science Experiments for Kids
- How to Grow Crystals
- Chemistry Projects
- Fire and Flames Projects
- Holiday Science
- Chemistry Problems With Answers
- Physics Problems
- Unit Conversion Example Problems
- Chemistry Worksheets
- Biology Worksheets
- Periodic Table Worksheets
- Physical Science Worksheets
- Science Lab Worksheets
- My Amazon Books
Sources of Error in Science Experiments

Science labs usually ask you to compare your results against theoretical or known values. This helps you evaluate your results and compare them against other people’s values. The difference between your results and the expected or theoretical results is called error. The amount of error that is acceptable depends on the experiment, but a margin of error of 10% is generally considered acceptable. If there is a large margin of error, you’ll be asked to go over your procedure and identify any mistakes you may have made or places where error might have been introduced. So, you need to know the different types and sources of error and how to calculate them.
How to Calculate Absolute Error
One method of measuring error is by calculating absolute error , which is also called absolute uncertainty. This measure of accuracy is reported using the units of measurement. Absolute error is simply the difference between the measured value and either the true value or the average value of the data.
absolute error = measured value – true value
For example, if you measure gravity to be 9.6 m/s 2 and the true value is 9.8 m/s 2 , then the absolute error of the measurement is 0.2 m/s 2 . You could report the error with a sign, so the absolute error in this example could be -0.2 m/s 2 .
If you measure the length of a sample three times and get 1.1 cm, 1.5 cm, and 1.3 cm, then the absolute error is +/- 0.2 cm or you would say the length of the sample is 1.3 cm (the average) +/- 0.2 cm.
Some people consider absolute error to be a measure of how accurate your measuring instrument is. If you are using a ruler that reports length to the nearest millimeter, you might say the absolute error of any measurement taken with that ruler is to the nearest 1 mm or (if you feel confident you can see between one mark and the next) to the nearest 0.5 mm.
How to Calculate Relative Error
Relative error is based on the absolute error value. It compares how large the error is to the magnitude of the measurement. So, an error of 0.1 kg might be insignificant when weighing a person, but pretty terrible when weighing a apple. Relative error is a fraction, decimal value, or percent.
Relative Error = Absolute Error / Total Value
For example, if your speedometer says you are going 55 mph, when you’re really going 58 mph, the absolute error is 3 mph / 58 mph or 0.05, which you could multiple by 100% to give 5%. Relative error may be reported with a sign. In this case, the speedometer is off by -5% because the recorded value is lower than the true value.
Because the absolute error definition is ambiguous, most lab reports ask for percent error or percent difference.
How to Calculate Percent Error
The most common error calculation is percent error , which is used when comparing your results against a known, theoretical, or accepted value. As you probably guess from the name, percent error is expressed as a percentage. It is the absolute (no negative sign) difference between your value and the accepted value, divided by the accepted value, multiplied by 100% to give the percent:
% error = [accepted – experimental ] / accepted x 100%

How to Calculate Percent Difference
Another common error calculation is called percent difference . It is used when you are comparing one experimental result to another. In this case, no result is necessarily better than another, so the percent difference is the absolute value (no negative sign) of the difference between the values, divided by the average of the two numbers, multiplied by 100% to give a percentage:
% difference = [experimental value – other value] / average x 100%
Sources and Types of Error
Every experimental measurement, no matter how carefully you take it, contains some amount of uncertainty or error. You are measuring against a standard, using an instrument that can never perfectly duplicate the standard, plus you’re human, so you might introduce errors based on your technique. The three main categories of errors are systematic errors, random errors , and personal errors. Here’s what these types of errors are and common examples.
Systematic Errors
Systematic error affects all the measurements you take. All of these errors will be in the same direction (greater than or less than the true value) and you can’t compensate for them by taking additional data. Examples of Systematic Errors
- If you forget to calibrate a balance or you’re off a bit in the calibration, all mass measurements will be high/low by the same amount. Some instruments require periodic calibration throughout the course of an experiment , so it’s good to make a note in your lab notebook to see whether the calibrations appears to have affected the data.
- Another example is measuring volume by reading a meniscus (parallax). You likely read a meniscus exactly the same way each time, but it’s never perfectly correct. Another person taking the reading may take the same reading, but view the meniscus from a different angle, thus getting a different result. Parallax can occur in other types of optical measurements, such as those taken with a microscope or telescope.
- Instrument drift is a common source of error when using electronic instruments. As the instruments warm up, the measurements may change. Other common systematic errors include hysteresis or lag time, either relating to instrument response to a change in conditions or relating to fluctuations in an instrument that hasn’t reached equilibrium. Note some of these systematic errors are progressive, so data becomes better (or worse) over time, so it’s hard to compare data points taken at the beginning of an experiment with those taken at the end. This is why it’s a good idea to record data sequentially, so you can spot gradual trends if they occur. This is also why it’s good to take data starting with different specimens each time (if applicable), rather than always following the same sequence.
- Not accounting for a variable that turns out to be important is usually a systematic error, although it could be a random error or a confounding variable. If you find an influencing factor, it’s worth noting in a report and may lead to further experimentation after isolating and controlling this variable.
Random Errors
Random errors are due to fluctuations in the experimental or measurement conditions. Usually these errors are small. Taking more data tends to reduce the effect of random errors. Examples of Random Errors
- If your experiment requires stable conditions, but a large group of people stomp through the room during one data set, random error will be introduced. Drafts, temperature changes, light/dark differences, and electrical or magnetic noise are all examples of environmental factors that can introduce random errors.
- Physical errors may also occur, since a sample is never completely homogeneous. For this reason, it’s best to test using different locations of a sample or take multiple measurements to reduce the amount of error.
- Instrument resolution is also considered a type of random error because the measurement is equally likely higher or lower than the true value. An example of a resolution error is taking volume measurements with a beaker as opposed to a graduated cylinder. The beaker will have a greater amount of error than the cylinder.
- Incomplete definition can be a systematic or random error, depending on the circumstances. What incomplete definition means is that it can be hard for two people to define the point at which the measurement is complete. For example, if you’re measuring length with an elastic string, you’ll need to decide with your peers when the string is tight enough without stretching it. During a titration, if you’re looking for a color change, it can be hard to tell when it actually occurs.
Personal Errors
When writing a lab report, you shouldn’t cite “human error” as a source of error. Rather, you should attempt to identify a specific mistake or problem. One common personal error is going into an experiment with a bias about whether a hypothesis will be supported or rejects. Another common personal error is lack of experience with a piece of equipment, where your measurements may become more accurate and reliable after you know what you’re doing. Another type of personal error is a simple mistake, where you might have used an incorrect quantity of a chemical, timed an experiment inconsistently, or skipped a step in a protocol.
Related Posts

IMAGES
VIDEO